Fraud analytics: What, why and its benefits
Fraud analytics is a broad term referring to strategies and data analysis for investigating and analyzing fraud. This article will help you know why fraud analytics are crucial to identify and mitigate fraud and how it applies to real-life cases.

Thulasi
June 25, 2024 |
7 mins
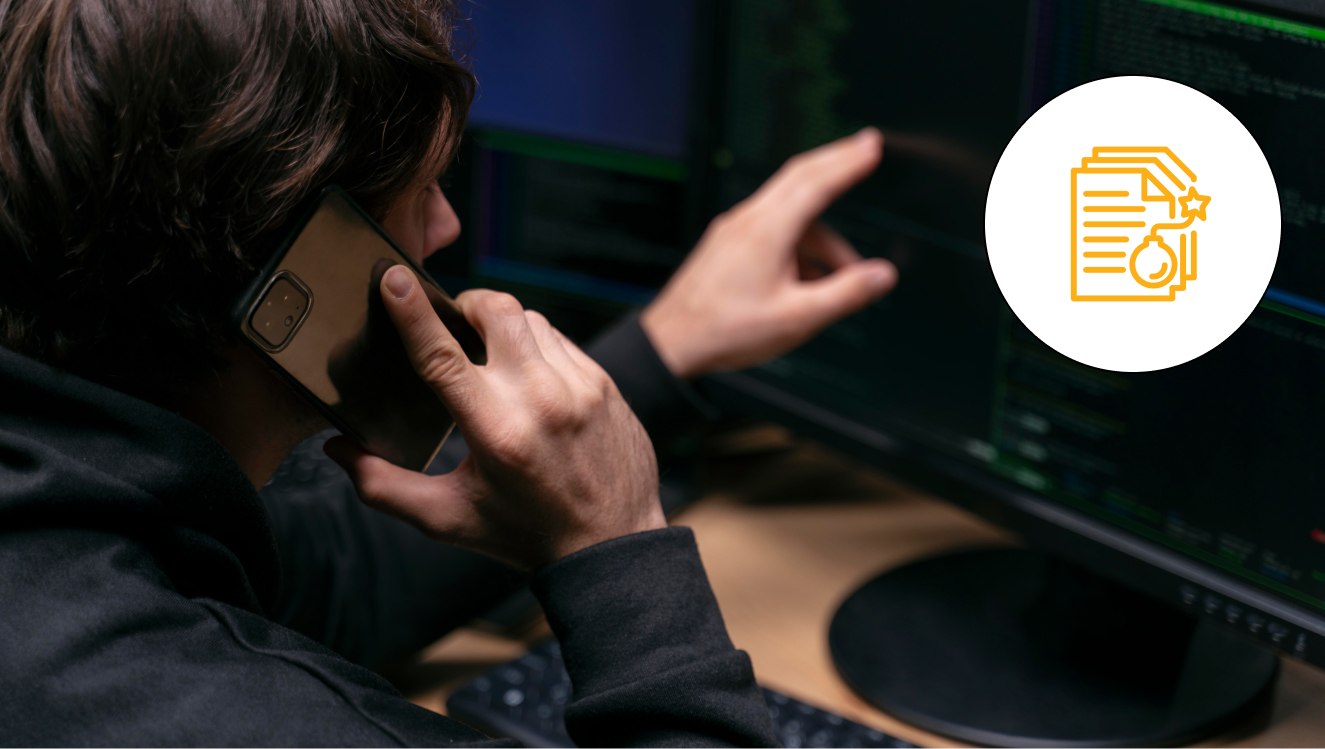
What is fraud analytics?
In sectors like banking, insurance, or finance in general, fraud analysts process a huge chunk of data and look for patterns and trends and identify anomaly behavior. For example, a banking customer trying to process a heavy payment from a foreign location could be suspicious. Allowing that to happen could be dangerous to both the bank and the customer. Identifying such activities in real-time through data mining, statistical analysis, and machine learning to flag or prevent them is called fraud analytics.
With the help of machine learning and AI-based algorithms, businesses can handle large amounts of data, predict, and prevent fraud in real-time. Fraud analytics is crucial for modern businesses to fight issues like money laundering, phishing scams, credit card fraud, duplicate insurance documents from customers and many similar issues.
AI and ML in fraud detection
Assume the number of transactions a financial institution processes and approves or think of the number of documents they have to validate. Is it possible for a fraud analyst team to monitor them? Firstly, it could become cumbersome and time consuming and, secondly, there could be false negative instances where a genuine customer could get affected.
Also, many companies are using rule-based methods and manual analysis to identify fraud. But, that’s not sufficient as fraudsters constantly change their strategies to bypass these rules and not get caught. Many modern tools and techniques are in their favor, and it is impossible to create and validate so many rules. That’s where AI and ML can help prevent fraud detection through the following steps.
It helps with data mining of millions of data and studying underlying patterns.
Compares and validates every instance across predefined rules to discover suspicious events that fall out. Can accommodate more rules as fraudsters develop their patterns and become more advanced.
Upon identifying a potential fraud, flag or intimate a fraud analyst to investigate further into the instance, reducing their workload as they only have to review selected scenarios. It could also raise alerts to the respective customer upon identifying such malicious activities. Example: signing in from a different device, multiple incorrect password attempts, etc.
AI could also analyze customer habits and behaviors and compare and score each risk instant.
AI-based fraud detection models improve with time and data being fed, improving its accuracy.
Techniques used for fraud detection
There are many AI-based fraud detection techniques. Following are some of the prominent methods used for fraud detection.
Predictive analytics
Predictive analytics is a commonly used technique to identify potential risks and happenings in the future by analyzing data from the past and learning the connections between variables.
The historical data includes customer account and transaction details, purchase history, previously committed frauds, claims, etc. Using machine learning models like random forest, logistic regression, or neural networks, you could score risk levels and label transactions as fraudulent and non-fraudulent. The model can identify and cluster accurately as it is already trained with labeled fraudulent data.
Predictive analytics is suitable for detecting credit card fraud, insurance claims, and eCommerce chargebacks. This technique could be effective as it gives warnings ahead of time for timely risk mitigation.
Descriptive analytics
Descriptive analytics is looking back into the past and understanding what has happened. It can be more of an in-depth investigation than prediction of a future event. Once it analyzes past data from different points, it could present its findings in a form of summary or visual reports to aid decision making. Visualizing the transactional and fraud data, businesses could understand common traits and come up with preventive measures. Descriptive analytics could also serve as a foundation for investigating suspicious behaviors that happened in the past and make an informed decision. To perform descriptive analytics, you will aggregate data from different sources. Upon applying statistical analysis, you could visualize them with the help of reporting tools.
Rule-based analytics
Rule-based analysis is a set of static procedures that verifies an instance against predefined rules. It is suitable for validating events and promote quick decision-making. Example: Approving a new customers’ bank account after evaluating simple parameters like age, salary, minimum balance, etc..
Unlike machine learning and AI models, rule-based analytics, it cannot identify or learn from the patterns within the data and adapt itself.
Rule-based system is suitable for simple workflows that wouldn’t require much scaling in the future, as introducing new rules can be quite tedious.
Pattern recognition
Pattern recognition is the foundational technique of all that studies and recognizes familiar patterns within data sets. It can quickly and accurately identify and separate the ones that match the patterns and the outliers. It’s used everywhere, from image recognition to OCR to fraud detection. Pattern recognition is suitable for fraud detection, as it involves transactional and behavioral data that share regularities. So, it’s easier to select features and train an ML model for outlier detection. This can be useful in identifying credit card frauds, fake chargeback complaints, fake eCommerce returns, and similar payment disputes.
Example of a pattern: how many payments a customer has made through their card vs. how many of them have been reported as a dispute?
Benefits of fraud analytics
Fraud and felony are everywhere around us, given the tech and internet evolution. It's affecting both customers and businesses, causing financial and reputational loss. See below to know how AI-based fraud analytics benefits businesses by fighting fraud.
Preventing financial loss
Global credit card fraud amounts to $33.45 Billion, which is equivalent to 6.5 cents per $100 of transactions and is predicted to rise further. It proves how fraud is a huge irrecoverable loss to financial service providers and innocent customers. Through fraud detection and mitigation, the number could be minimized and brought to control. For example, real-time alerts on suspicious high-value transactions could alert both the customer and the risk team to look into it and block that on time. Or, banks could prevent a fraudulent chargeback which otherwise would have gotten approved.
And that’s how businesses could be wary of potential fraud cases and save money for them.
Other than ensuring high profitability, financial institutions could also build strong relationships and trust with customers, keeping bad actors away.
Reducing workload for risk teams
By automating risk management, your risk team could handle higher workloads at faster rates. Rather than evaluating every reported transaction and fraud, they could only review handpicked events by the fraud analytics system. Our datakulture team created a similar fraud analytics system for an NBFC insurance seller. Now they have improved their fraud detection rates of claim requests, and approve requests faster. Their workload is more streamlined and they are prepared to scale better without having to expand their team rapidly.
Chargeback fraud
Ever notified your credit card to your payment network provider of a fraudulent charge on your account for a product you never purchased? That’s chargeback and it’s the responsibility of your bank/financial institution to investigate and refund the money. In many cases, these chargebacks are legitimate requests where the customer is not aware of the merchant withdrawing the payment. But there are some customers who take advantage of the situation, make a spending, and then report that someone stole their card. More than the financial institution, the retail, SaaS, or eCommerce merchant becomes the victim here as they lose their revenue and even pay penalties. Imagine delivering a product or service without the customer paying for it.
Fraud analytics and pattern recognition could help financial institutions put an end to these fake chargeback reports. By looking into customers’ past purchase data, reported chargebacks, and other data, banks could make informed decisions and prevent loss for merchants.
Near real-time monitoring
Millions of transactions happen all over the world over a second. The power of real-time and near-real-time monitoring can make a lot of difference in preventing fraud and felony. It’s much easier to prevent something rather than investigating them in the future, wasting time and resources.
AI-based fraud analytics in banking empower them to do this, having control over near-real-time transactions. Be it a small chargeback or a huge money laundering activity, the respective institution can monitor that in real-time, look for suspicious activities, alert and block on time.
Adapt to evolving fraud patterns
Fraudsters and imposters change their patterns and tricks over time and use advanced tech to their advantage. When you use standard rule-based systems, you will not have many options to develop your systems’ knowledge base to capture these evolving crimes. Machine learning based algorithms are versatile on the other hand. They can continuously learn from their data and improve its detection rate, simultaneously helping the risk team to re-evaluate their risk scores.
Final thoughts
Every day and every minute, there are tons of fraud and imposter cases being reported all over the world. Customers are wary of this now and look for brands and financial institutions with higher reputation and stronger mitigation strategies. That being stated, fraud analytics become an inevitable investment for growing businesses to secure their competitive edge and earn customer trust. Investing in a robust and advanced fraud analytics system is the need of the hour for long-term success in this complex landscape.
With more machine learning and data science solutions in the market, the picture is as clear as muddy waters for decision makers on what to choose for risk assessment. If you are one of them wanting to equip them with high detection rate systems, reach out to us. Having built risk and fraud analytics solutions for financial institutions, we can help you develop solutions tailor-made to your requirements.