Machine learning OCR: Everything you need to know
The blog is packed with three parts - how OCR works, the role of machine learning in OCR, and its advantages and use cases across industries. Read the full blog to know more about how OCR and AI are becoming a powerful combination and making strides in accurate text recognition.

Thulasi
May 3, 2024
|5 mins
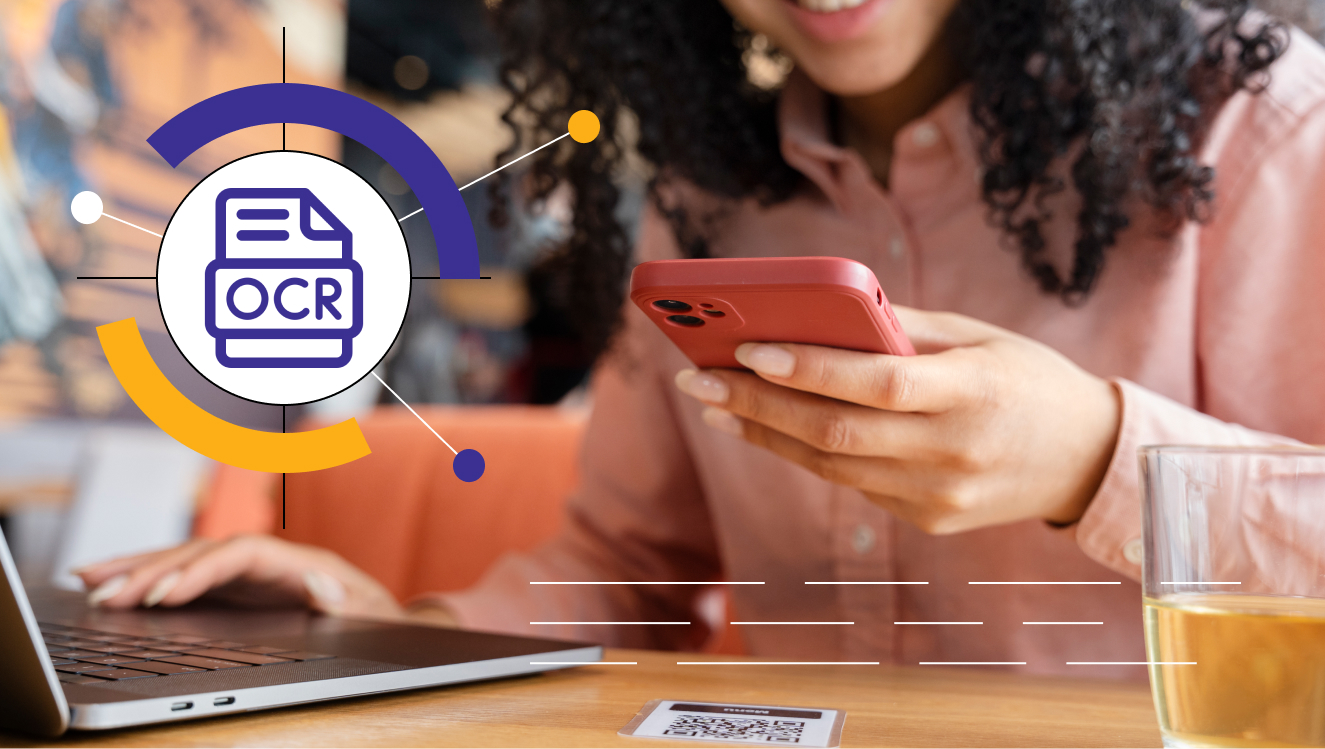
What is OCR?
OCR, optical character recognition scans and reads handwritten, printed, or digital text and converts them into digital format. In pure business terms, it’s making the unsearchable into searchable documents. Imagine a document entry process where you manually enter fields onto a digital system. OCR cuts this chaos, allows you to scan the document, and auto-fill the entries, allowing you to edit if necessary. Or remember the time you understood a billboard in an unknown language by scanning it through translation apps? Technologies like optical character recognition and text recognition power all these. From automatic license plate recognition to data entry tasks, you can find its applications in every field.
So if you ask whether OCR is artificial intelligence-based, here’s your answer. Traditional OCR systems rely on machine learning, hidden Markov, or other statistical methods to capture texts and convert them. However, not every text capture comes in an ideal, flawless format. So, modern OCRs use artificial intelligence like deep neural networks which improves their capabilities—allowing them to deal with semi-structured data, and process any type of document with accuracy—like intelligible, unclear, or wrinkled handwritten documents. It can even recognize the information it’s capturing and perform post-processing on its own, minimizing human interference.
How does OCR work?
Typical OCR involves three processes.
Pre-processing: this is where the target area with texts is scanned. The scanning system tries aligning the text to be captured in line with the scanner’s input, zooming in and out. Once the target area is captured, it’s smoothened to remove any foreign particles that affect the quality of the image, any overly-exposed dark areas, marks, or blemishes.
Then, it undergoes binarization where the image is converted into a black and white image - black representing the text areas and white is set for anything other than texts. The image is also re-sized to accommodate the right size required for text extraction, which is 200 to 600 DPI.
Character recognition: The next step is character recognition. This involves utilizing AI-based technologies like Convolutional Neural Networks which segments text into individual characters and feed them to the machine learning algorithm.
You label images of text and train the model on them, helping the model associate visual features (like curves and lines) with corresponding characters. Based on what the model learns, it produces a probability distribution for the test data, shares possible characters for each segment, and selects the highest probability for the same.
Post-processing: Summing up, OCR splits up an image into text and the rest, breaks down the text into words and characters. The ML algorithm recognizes characters, combines them, and presents the output on the screen. Post-processing also includes removing misrepresentations of words and enhancing the accuracy of the recognized text. For this, the algorithm is trained with loads of vocabulary to understand how words are written.
This rapidness and accuracy in conversion make machine learning more suitable for text recognition, increasing the demand for OCR based on artificial intelligence.
OCR use cases across different industries
We see and use OCR every day even without realizing that working in the backend. Here are some common and uncommon use cases of that.
Banking
From handwritten checks to manually filled-out forms, banking stays on top in OCR utilization. They are also catching up on digitization, converting heaps of files and reams of documents into digital records. This industry also uses OCR for document validations. So, no employee will be wasting time on meticulous inspections as AI-powered systems handle data extraction and comparison.
If integrated with their key banking applications, OCR can be vital in improving efficiency and preventing fraud.
Insurance
Strict regulations and document-heavy workloads don’t always go hand in hand. It requires proper document management practices to do this right. But, there are also bundles of client files, proofs and certificates, policy forms, and other legal papers existing in physical format. This brings OCR into the picture to sustain its digitization efforts without overburdening customers or employees. Combined with artificial intelligence, they can even generate frequent data-driven insights on customer behavior, demographics, and more.
Healthcare
This industry deals with tons of manual documents - from patient notes to hospital management records to bills. The complicated industrial terms and intelligible handwriting make data entry tasks harder. This is where OCR can help, scanning documents on the go, and creating an accurate digital library. artificial intelligence-based OCR can go a level further, and tag relevant files based on the information it contains.
Logistics
The world of consignments, vehicles, and packers uses OCR in many ways. From scanning the license plate of vehicles for tracking to extracting information from invoices, they have many OCR use cases integrated into their warehouse management systems. An employee dealing with inbound delivery can scan its label and auto-register its details. It also powers advanced use cases with the help of AI, further aiding automation. For example, the OCR can scan a consignment and share inferences on how to handle, storage slots required, delivery instructions, or anything it’s programmed to perform. These minute changes can exhibit significant delivery time, helping logistics companies maintain good relationships with their vendors and clients.
6 advantages of OCR for your businesses
OCR helps many industries and powers many use cases. How exactly does this technology help you?
It saves time and cost
Employees are freed from tasks they fear - manual data entry. OCR lets them work smart, requiring only minimal interference. This is not only time-saving but money-saving too.
Precise data
Modern OCRs are adaptable and can scan any kind of document, be it in any format, and still reap information with full accuracy. With, trained machine learning-based OCR, you don’t have to worry about frequently correcting its outputs.
Improve productivity
As employees don’t have to waste time on inputting numbers and characters, they can focus on getting their actual work done. A person working in a bank doesn’t have to scrutinize a manual signature for minutes and let the OCR validate it against their original signature.
Improved data quality
Data is extracted with 99 to 100% accuracy without room for human errors. So, you can store data without further cleansing or pre-processing. Besides, you will not be stuck with scattered data, half on cloud storage and half on physical files.
Compatibility
Modern OCR systems give you power and flexibility by offering you multiple options for generating the output. They support multiple digital forms like PDFs, docs, spreadsheets, images, text files, or any other searchable formats. You can extract and load the information in any of the above ways, process it, or save it for further usage.
Centralizes your data
OCR technology gives you a way to centralize your data storage so you don’t have to safeguard any physical documents. This can fuel any analytics or business intelligence projects you are running without excluding any data chunk. A quick example of this is scanning physical invoices from vendors, extracting exact information using machine learning, and adding that automatically to your accounts payable. This not only gives you a way to auto-schedule payments on time. It also allows you to dive deep into your recent spending, project future expenses, and strategize ways to improve ROI.
Summing up
The history of OCR dates back to 1968 when the first OCR device was invented with simple scanning and extraction capabilities. Ever since there have been monumental strides with the recent ones being the integration of artificial intelligence technology. What used to take hours now only takes seconds, capturing what needs to be. It can be integrated into any product for use cases like invoice capturing, document extraction, and many other process automation. You can do this too, reforming how your employees work while digitizing on the go. Now let’s get to how to layer your workflow with machine learning or AI-powered OCR technology.
You have many open-source and enterprise products available like Tesseract, Nanonets, Datacap from IBM, etc. It can take up to a few weeks to set this up and tune as per your requirements. This is particularly true when you need a specific use case built around the Optical character recognition technology. Your model has to be trained against your data format so it works seamlessly. This training data has to be prepped and standardized. The right OCR tool suitable for this use case should be chosen as well. All of this requires a team of data scientists who grasp how this application works for your organization’s end users, either employees, vendors, or customers.
Enter datakulture with data professionals brimming with up-to-date skillsets and real-world experiences in building OCR-based machine learning systems. Fix a call with us to hire the right specialist who can make this a breeze for you. Delve into our case studies to weigh up our team’s exposure to modern-world data concerns.
Say no to human intervention errors
Get in touch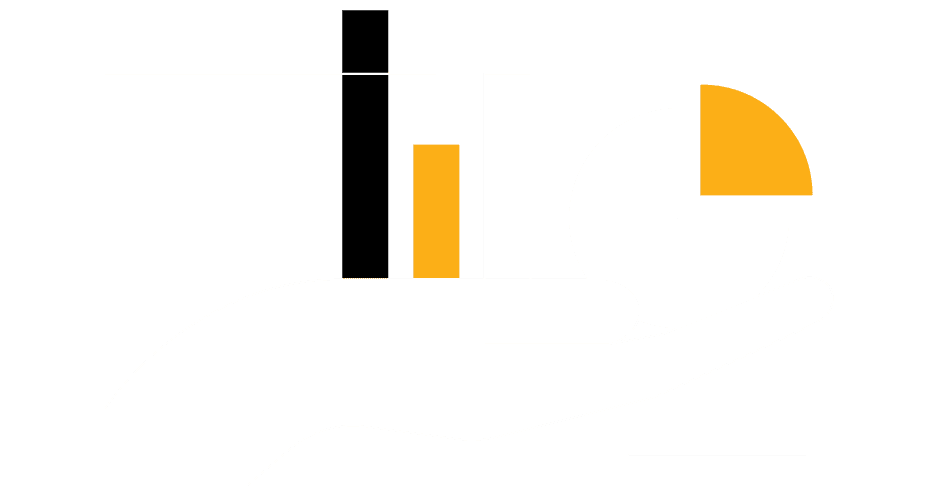