Augmented analytics - Definition, use cases, and best practices
How can business users get faster access to insights from their data without interference from data professionals? Augmented analytics is all about that and can level up your decision-making process offering deep, helpful, otherwise hidden insights. Read the blog to learn more about making this a part of your data strategy.

Karthik
May 9, 2024
|7 mins
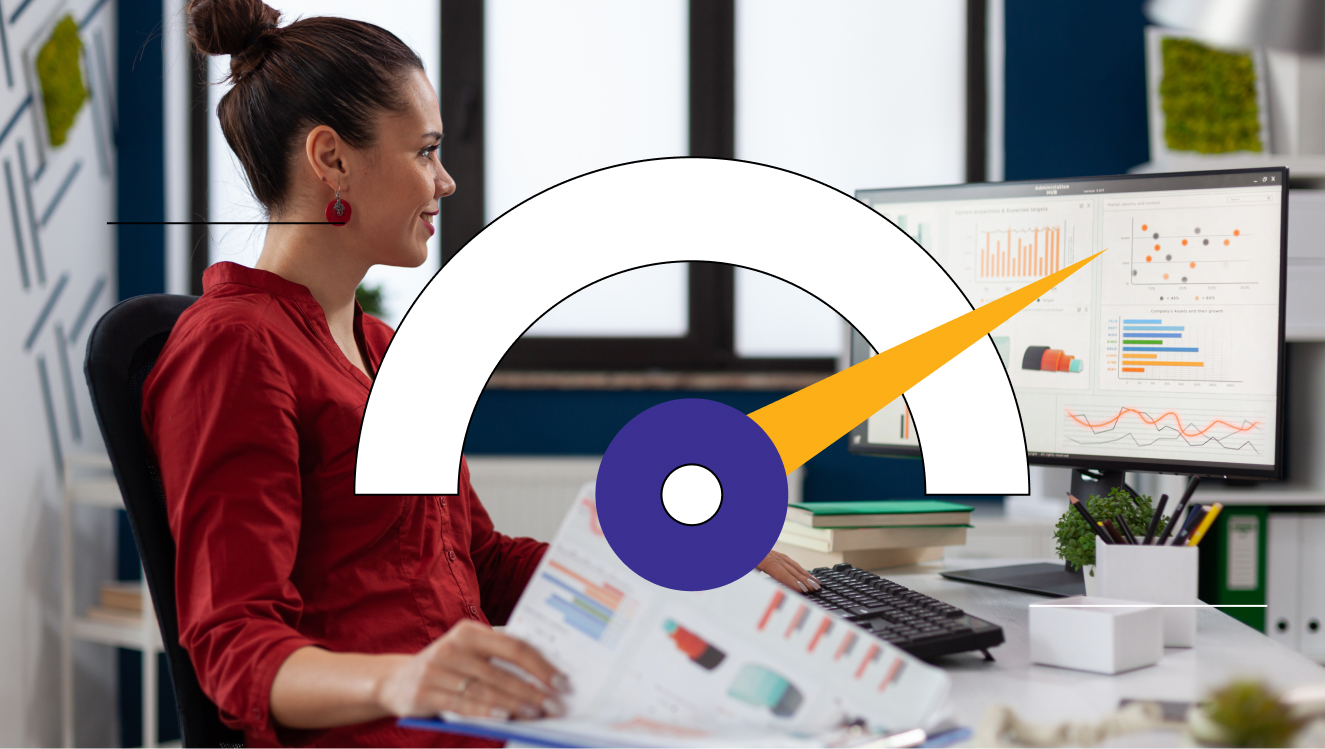
What is augmented analytics?
Traditional business intelligence often confuses business users with too many variables and data points, delaying them from making the right decisions. Plus, a data team sits in between, preparing data for reporting which further delays the process.
While self-service analytics bridges the gap between business users and data, the decision-maker is still responsible for extracting critical insights and applying them on time.
Augmented analytics elevates this and amplifies the data analysis process, using AI-based technologies like NLP and ML. So, business users are automatically offered the best next steps, recommended actions, and hidden trends to make quick decisions.
Key components of augmented analytics
We have seen artificial intelligence as the major driving force behind augmented analytics. Here is a quick breakdown:
Machine learning
We have already seen that augmented analytics offers detailed insights from your data that business users can instantly act on. This is possible with machine learning algorithms that extract trends and insights from data and dish them out to users on time. We can take a recommendation system of a streaming platform for example. It analyzes users’ preferences, watch history, likes, and dislikes, and suggests shows that they might like. It’s up to the user to watch or rate the accuracy of the recommendations. Similarly, ML algorithms share correlations for leaders, necessary for them to make critical decisions.
These algorithms analyze data collected from various sources, each in different formats, slice and dice to reveal hidden patterns, and contextualize and visualize them in the form of recommended actions.
Natural language processing
In traditional BI, data experts are needed as mediators so users can communicate with data processing systems. NLP becomes a bridge here, allowing users to directly interact with systems in natural language.
With a conversational UI, users don’t have to reach out to the IT or data team, whenever they need data. They can ask questions and receive answers from the analytics system or a platform where augmented analytics is embedded.
NLP becomes the core, interpreting human language to the system and vice versa. It also summarizes what the ML algorithms found contextually so users can grasp it quickly.
Data visualization
These are tools that turn content-heavy data and information into comprehensible visuals. Visualization is the key to escaping the higher amounts of data while satiating the quest for insights.
Voluminous data is converted into easily consumable and digestible visual representations such as scatter plots, histograms, treemaps, bar charts, etc for quicker absorption.
Augmented analytics benefits
Augmented analytics is the pathway to a new era of decision-making. Here is how it helps businesses like you.
Make data-driven decisions
Being able to get filtered information whenever someone needs it, in a format you can understand enables faster decision-making. This puts you business leaders in the front seat, saving their valuable time lost in guesswork or communication delays. This is how high-growth companies thrive - they turn to their past data to craft their future, picking every minute trend and tendency and acting lightning fast.
Detailed insights in real-time
You don’t need a data scientist to analyze and summarize detailed insights for data consumers. Augmented analytics automates this - providing answers to not just for what’s, when’s, and why’s but also how’s.
These insights are enough to come up with an informed conclusion without seeking additional information.
More accurate data
Erratic data recording = unreliable and misrepresented insights. These human errors can cause a skewed representation of the past, leading to miscalculated decisions. Augmented analytics lets you overcome this. Since it’s fully automated, the risk of human errors is minimal, promising you accurate data.
Generate more user-specific reports with smaller data teams
As seen earlier, augmented business intelligence cuts down the reliance on IT and data teams. At the same time, you will not be depriving your stakeholders of the necessary figures they need for running everyday errands. Artificial intelligence meets human inquisitiveness, and feeds them with relevant answers, scrutinizing information that have authority over.
Think of this as your organization’s magic box that fetches answers in seconds in the natural language.
Augmented analytics use cases
Augmented analytics is for everyone. It offers applications that cater to various industries, functional areas, and decision-makers.
Marketing and sales
Living and breathing data every day, marketers and sales professionals can benefit from augmented data management. They need frequent, trench-level insights on different factors to be on their marks anytime.
For instance, a salesperson can identify and pick accounts likely to convert based on insights received. A marketer can understand the most profitable mediums and channels, why are they so, and find better strategies to focus on that. Or, they can understand how dynamic pricing works for their business and employ optimal pricing strategies.
They can do this and more - without spending hours crunching numbers, analyzing reports, or cross-checking different systems.
Entertainment, streaming platforms, and media
The growth of music and video streaming platforms has been exponential. So as their dependency on data. With more users tuning to a wide variety of content, these companies are left with data—lots of’em. The art of managing the humungous data and the quest for reliable insights underscore the importance of augmented analytics. With amplified analytics, the industry can,
Find out the best-performing content, label, and genre for each group of users, demography, age group, and other characteristics. These stats can help them view what works for whom and match the right audience with the right content.
Tune their marketing campaigns the right way with the optimal suggestions shared.
Devise strategies for reducing customer churn—a growing concern for this realm—by knowing what satisfies their customers.
Healthcare
One industry that’s slowly adopting data-driven strategies is healthcare. They can amplify their efforts with augmented business intelligence this way.
Predict disease spread and outcomes way ahead, so they can act faster and save lives.
Understand patient and disease outcomes and receive the next course of action without wasting time on documentation or data analysis.
Handle hospital operations better - from allocating the right number of staff or bedding to expense management to inventory tracking.
Implement a system where anyone can ask hospital-related questions and find answers - like availability of doctors, appointment-related questions, and more.
Manufacturing
If there is one sector that must be entirely forward-looking, it’s manufacturing and supply chain. While there are many AI use cases for manufacturing, augmented analytics hold an important spot.
They can be demand-driven if they employ augmented data management practices. Here are some augmented analytics examples that will be helpful for this industry.
Receive in-depth demand forecasting trends in real-time, something that changes every minute and is dependent on multiple external factors.
Use predictive maintenance to reduce unnecessary downtimes for machinery.
Augmented analytics best practices
It can take a while to switch from regular business intelligence to augmented analytics. To ensure you receive effective results and long-term benefits, follow the steps below.
Establish your foundation and align with your KPIs
A step-by-step approach is well suited for any BI activities. This is advisable as you cannot become data-driven in the short term and expect an instant culture change within your organization.
Look back and observe your current analytics process. How does that work? What are its flaws? Where do users need instant access to data? Ask questions like this.
Start small and start laying the foundation for a robust, advanced BI, keeping security and governance in mind.
Initiate with a modest scope and lay the groundwork for an, advanced BI infrastructure, keeping in mind the security and governance requirements.
Get some success and scale to AI analytics
Rather than going all-in on AI, pick any part of your company and test how AI is augmenting analytics there. Note down the results. The difference between the past and current process should tell you if augmented analytics is for you.
Demonstrating small successes and scaling it organization-wide should be your motto for this initiative.
Collaboration
Augmented analytics isn’t here to isolate business users from data experts. It should rather strengthen their bonds while smoothening everyone’s work. Help your data scientists grasp their new responsibilities. Concurrently, allow non-data people to experiment, learn, and ace analytics along with data literacy.
Data governance
This holds utmost importance just as the above measures. It’s about how you utilize data to its fuller extent while ensuring its security, accessibility, quality, and privacy.
Hence, this should be the blood and backbone of your analytics engine. Let it evolve as you move from regular to augmented intelligence. Let it dictate how data is managed across your organization, throughout its lifecycle.
Conclusion
If you are sure augmented analytics is your next stop, you have two options to start with. Try your hands on out-of-the-box applications for analytics or get your custom analytics solution built - launching it as a standalone insights delivery platform or embedding it as a part of another application.
If you want to understand how this can be useful as you scale, send us your message. We can explain how a custom-built analytics solution works, where to start, what to measure, how to scale, and everything in between.
You can also refer to a case study that demonstrates how we have set up a one-stop analytics solution for one of our clients.
Analyze, visualize, and get insights from data
Get started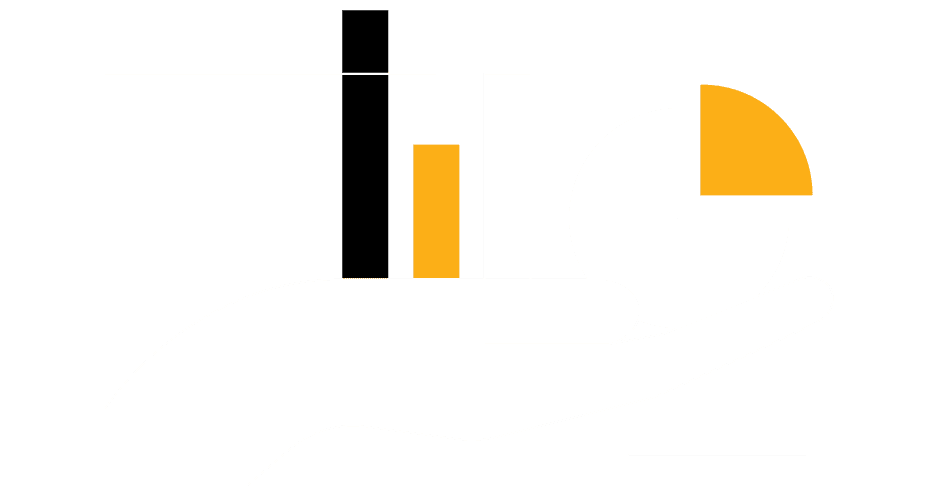