Self-service analytics: Best practices, challenges & benefits
What if everyone in your organization is equipped with the data needed to make their own decisions? This concept is called self-service analytics. Read the full blog to know its benefits, challenges, and how you can set this up.

Surendar
April 26, 2024 |
7 mins
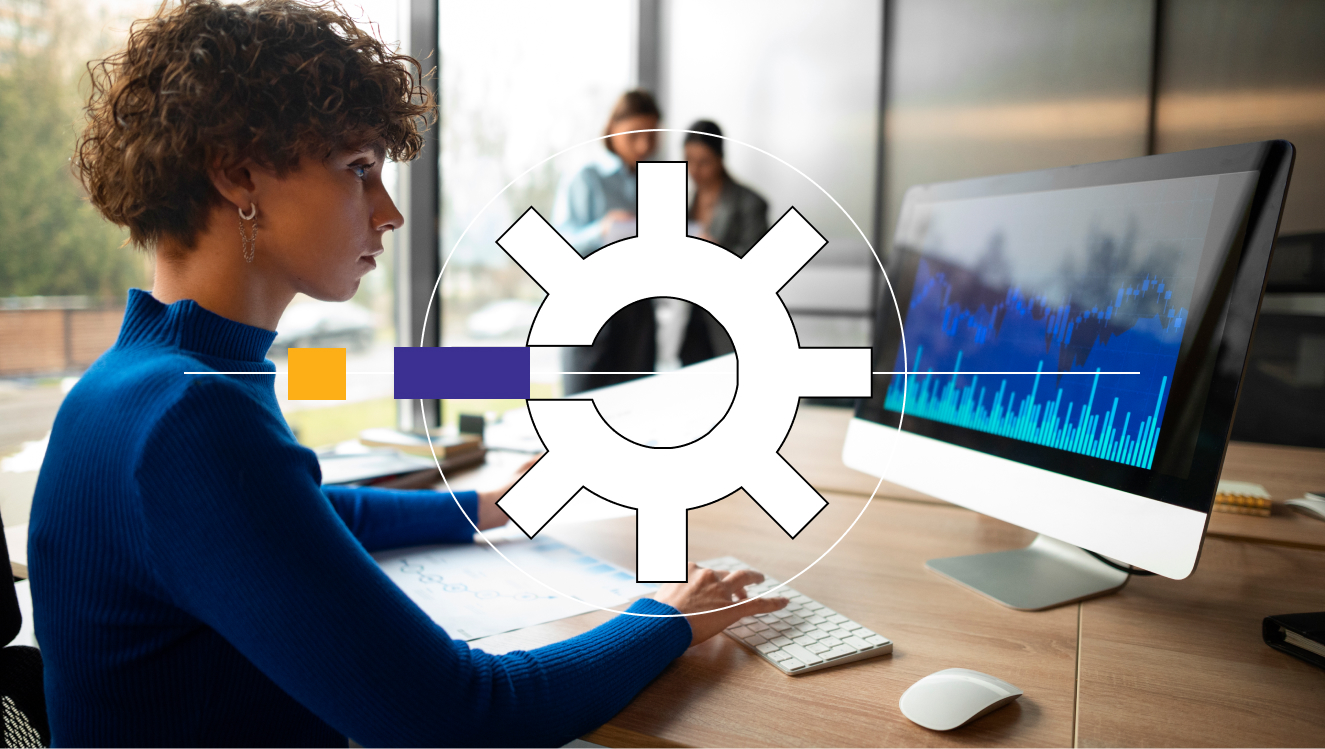
Importance of self-service analytics
Organizations are becoming increasingly people-centric, breaking siloes and barriers, allowing everyone to contribute to the decision-making process. But where do they get all the information needed to make the right decision? That’s where organizations step in, and enable self-service analytics platforms, so the right information reaches the right person at the right time.
Otherwise, the data practices in the company would remain IT-centric, taking care of everything from report generation to insights delivery to the business stakeholders.
A decentralized approach is what contemporary organizations need where end users and leaders make instant yet informed decisions - without having to wait for the IT team. It’s a next-level business intelligence that slices and dices data to suit everyone’s analytic requirements, making it available at their fingertips, quite literally.
The following sections discuss how to implement a decentralized approach along with strategies for avoiding common hurdles like governance.
Self-service analytics best practices
You have decided self-service data analytics is your next mission. How to go about it? How to avoid classic pitfalls that many are bound to encounter?
Follow the steps below.
Identify your needs
The best way to map out needs should start by asking consumers what they want to achieve. Here the consumers mean your teams, departmental managers, and other key personnel. Find current challenges they face with accessing data and reports and how they want them to be improved.
In terms of data, what are the questions they typically try to derive and what key insights do they require from time to time?
What are the frequent misconceptions and extrapolations occur in their processes due to the non-availability of these data?
Finding answers to these questions is a good start to lay the foundation for a strong self-service analytics strategy.
Choosing the right platform
Once you know what data they want and how they want to access them, you can find a suitable business intelligence platform. There are many plug-and-play analytics solutions available in the market for this. Examples: Tableau, Qlik, etc. They acquire data from various platforms and present them in the form of visualizations, allowing users what they can access.
With the help of an expert data visualization team, you can implement this self-service analytics platform.
When you select the tool, make sure you check the following factors.
Whether the cost of the tool balances with the benefits and values it offers
Reporting and visualization capabilities
How it aligns with your organization’s needs and provides the most needed functionality without overwhelming with not-so-required features.
Whether it connecting as many data sources as possible seamlessly
Where does it operate - cloud or on-premises?
Whether the tool supports natural language queries or requires SQL knowledge to get answers.
📖 Recommended read: Augmented analytics
Data governance
Self-service analytics might pose heavy data leakage and governance issues which require devising user roles, access policies, and your organization’s standards for data analytics.
Imagine someone from the sales team having access to critical data from the finance team. This can be threatening to your organization’s data security and your IT team wouldn’t be happy about this. On top of that, there are strict compliances like HIPAA, GDPR, etc which your organization must adhere to.
The process starts with planning who needs access to what and on what levels.
Your users should also have an understanding of the data they access through self-service analytics platforms, not just its business context, but where it comes from, how to query and share reports, their limitations, and more.
Simply put, you are empowering users to access data as they go, but with certain restrictions and constraints.
Build it, test it, and scale it
Self-service analytics is definitely not a one-day project. You need to find out how your team adapts to this new system. At the same time, you cannot overwhelm your data team to set up and generate 100s of reports from day one.
It’s advisable to build your self-service business intelligence platform first, connect the most essential data sources, and test how it works. Observe the value it adds to your company and how it quickens the decision-making process.
Collect feedback from relevant teams, make necessary UI and data integration changes, and repeat that as an iterative process.
Slowly, you can start scaling, add more data sources, and set up self-service analytics across the organization.
📖 Recommended read: Business intelligence in retail
Know your limitation
You cannot make every ounce of data available through self-service analytics platforms. Certain data isn’t cut out for this simply because of the following reasons.
It might require too much cleaning or pre-processing but might serve very little value.
Might be too sensitive to share with business users.
Might be not required for analytics purposes.
Analyzing this will help you remove the extra burden off your analytics platform without affecting the analytics process or exposing important data.
Self-service analytics benefits
Self-service analytics isn’t only useful to decision-makers but the whole organization. Here is how it serves value and return on investment.
Make better decisions
With rapidly changing economic, social, and business landscapes, leaders must make decisions lightning-fast. And these decisions cannot be instinct-based and rather be backed up by up-to-date data. Self-serve analytics is the only way to pass down these decision-making insights to these data-driven leaders. Whether it’s about the effectiveness of a marketing campaign or the lead conversion rate of a particular month, insights can be served to whomever it applies to.
Saves time and cost
Self-service analytics cut down the need to wait for hours to get answers to your business questions. The speedy time to insight enables you to make instant, effective decisions without depending on any specific data team. This also cuts down the cost of maintaining a team of data analysts and visualization experts who convert the raw data into applicable insights. Rather, the tool does the job automatically, making insights understandable for whoever it concerns.
Data accuracy
Accurate data is important for effective analysis and decision-making. Automated ETL processes ensure data accuracy, keeping null values, duplicates, and outliers away from the data. This can be also possible when you employ a team of data experts and use different tools for data preparation. However, the process has to be a little more elaborate and extensive (still cannot rule out the possibility of human errors).
Easily accessible to everyone
Data-driven culture should thrive from the bottom up in an organization. Self-service analytics works like a foundation for this, enabling everyone to handle data on their own, contributing to the organization’s success in ways they could by making potential calls accurately.
They can not just go through this data but have a sense of understanding of what’s happening in their organization, within and across their teams as well.
So, next time when you conduct team meetings, not just the presenter but everyone gets what these data points mean. This pays the way for a more inclusive participation from anyone.
Decreases workload for the IT team
When your employees can access data and generate reports on their own, they don’t have to rely on their IT or data team for their periodic reports. This reduces the burden from both ends, allowing the IT team to focus on more productive and strategic tasks. They can rather become the moderator and oversee the whole process.
Challenges of self-service analytics
Despite its benefits, there are still challenges with implementing and executing self-service business intelligence. It’s crucial to be aware of those before you begin experimenting with this concept.
Data literacy
This denotes the ability of users to understand, handle, and explore the data they have access to. Data literacy is a serious issue that businesses must keep in mind as without this, their data initiatives will not reap real value.
But why is this an issue?
The humungous amount of data a business handles can be seriously overwhelming at times to these users. Besides, not every self-service analytics platform is set up in a user-friendly, explainable way with metrics that really matter. All of this can lead to the following either procrastination of important tasks and decisions or misinterpretation or mishandling of data.
Fix? Conduct data literacy experiments, educate your users to become data literate, and help them develop tech(math, analytical, and programming skills) and non-technical skills (critical thinking, understanding connections, subject knowledge, etc) related to data.
No data governance
While you might set up governance policies from the start, it isn’t enough. Governance isn’t a do-and-be-done-with task. It requires continuous monitoring and frequent updates as your analytics goals take new turns.
If you don’t have a proper governance and risk mitigation strategy in place and don’t make it the base of your analytics efforts, then it can be a severe problem as you proceed with your analytics efforts.
Inconsistent data
Not every company properly collects data, especially when it’s about historical data. Data might be unevenly distributed with a few or many missing tables. This can be a hurdle when you want to run extensive analytics experiments considering the past and present data.
The good news is that there are many ways to handle these sparsely present, fragmented data. With a skilled data team, you can cope with this challenge while setting up a strong data foundation for the future.
📖 Recommended read: Data storytelling
Transitioning from a centralized to self-governed analytics
In a traditional business environment with a centralized IT setup, the IT team holds ownership over data and reporting processes, prepares reports, and shares with relevant business people. This centralized governance helped with protecting their sensitive information and overseeing who gets access to what - in an extremely vigilant manner.
And then there is the delegated governance model with roles growing beyond the IT team like data stewards and administrators. Here, the IT teams go beyond merely providing reports, enabling data consumers to access data sources. It’s like data consumers having their own sandbox environments to safely copy and save content from data sources. While the permissions granted are still limited here, this requires higher technical skills and transparent processes to be successful.
Then comes the mid-ground between both - the self-governing model. With a solid collaboration between the IT teams and data consumers, this model allows users to have access to certified content as well as data sources. Along with this, creators can also come up with ad-hoc reporting for their frequent business needs. But here the consumers can clearly understand the difference between certified and ad-hoc reporting with clearly defined validation and certification processes.
Eventually, companies with centralized analytics can transition into a self-governing analytics model where IT teams can decide and delegate a few responsibilities based on users’ analytics capabilities. This will organically move to the next level as users start asking more questions and want to find answers within their data themselves.
The proliferation of analytical skills among users from all backgrounds will also be a contributing factor here in switching business users to creator roles with appropriate permissions and checks.
Conclusion
According to McKinsey’s report, data-driven organizations are said to be 19 times more profitable than those that don’t. The right place to start with is democratizing data and making it a practice for everyone not just your top management. So, self-service analytics is the optimal solution for this.
Yet, with so many self-service analytics platforms, each with different features, you can easily get distracted and you will need expert help figuring out the right solution for your team. This is where datakulture can step in. Having implemented self-service analytics and modernized visualization for many companies, we have devised a successful approach to build your analytics strategy right from scratch. Another advantage is our team of experts who come with data skills and subject matter expertise, working with different industries.
They can understand your analytics needs, derive what you should measure, and devise the right solution. Send your requirements below so we can have a quick conversation on how this can work for your business.