Predictive analytics in marketing
Marketing team must be a step ahead of the company and its competitors. How can predictive marketing help marketers achieve this, predict the future, and stay ahead of trends is what the whole article is about.

Thulasi
Sep 25, 2024 |
7 mins
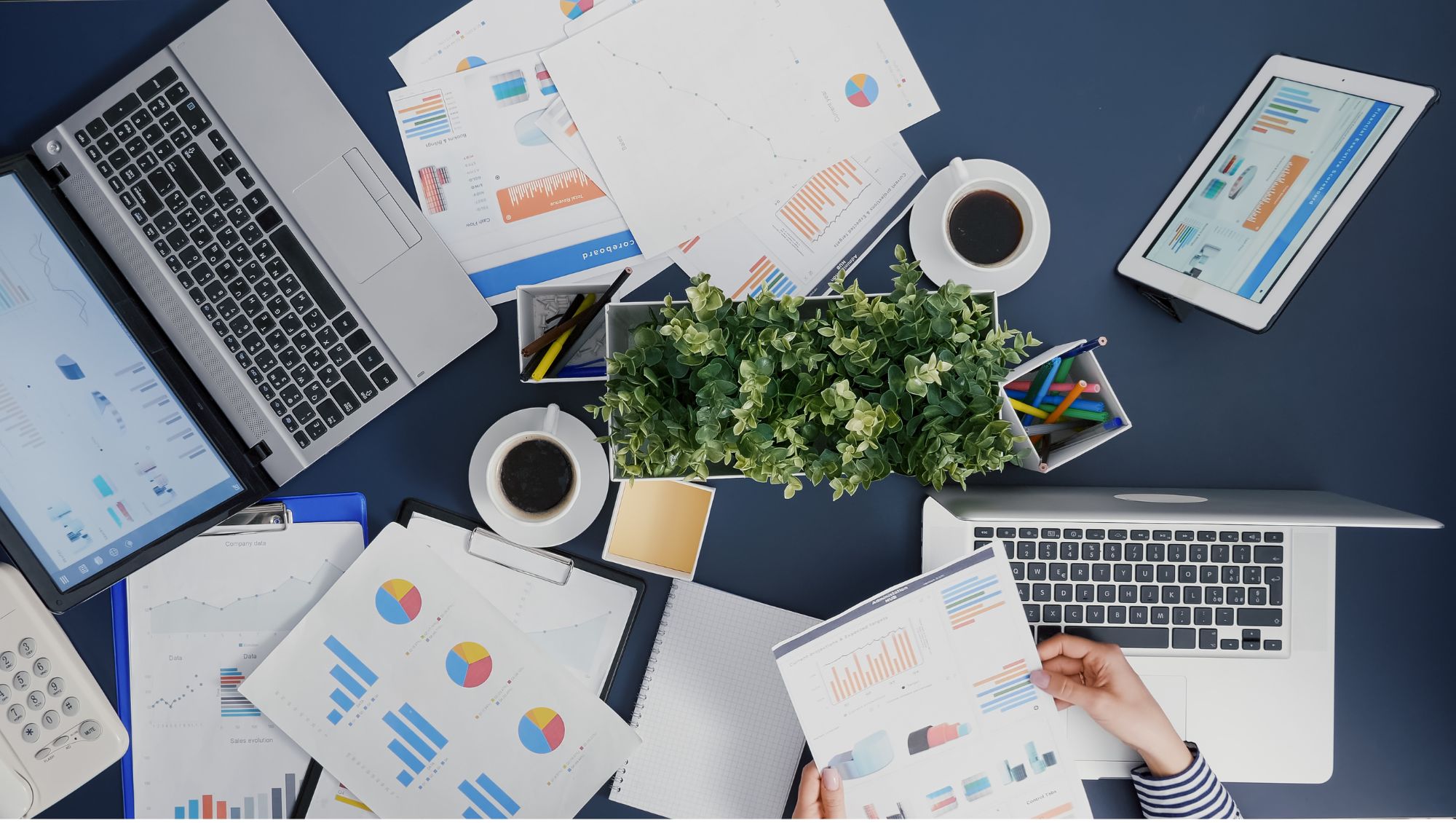
What is predictive analytics in marketing?
Predictive analytics in marketing is a statistical and machine learning approach which predicts future trends using historical data. With the power to see how customers are likely to react, marketers can tweak their campaigns, come up with better strategies, personalize messaging, and increase ROI.
Predictive analytics is an important tool for marketers as it helps them make sense of multiple streams of customer and campaign data. Manual analysis may not be possible in all cases, and even if you do, it's a lot of work and results may not be accurate.
That’s where predictive analytics help. Be it numerical or categorical values, it can process them all, null out outliers, and filter hidden trends that have useful messages.
With predictive analytics, marketers can
Aggregate data from various campaigns and sources and trace patterns behind.
Predict customer preferences and behavior even in a dynamic environment
By staying alert on market and customer trends, can stay prepared and proactive with planning and execution.
Do real-time adjustments to marketing campaigns to maximize results.
Stop making gut-based decisions and gain trust in data to make informed, ROI-improving decisions.
Predictive marketing examples in marketing
Predictive analytics is used in marketing for customer segmentation, personalization, demand forecasting, and churn prediction. It’s also used for optimizing marketing campaigns, lead scoring, recommendation system creation, and many similar marketing objectives. Companies like Google, Uber, Netflix, Nike, Spotify, and Starbucks are already using predictive analytics for marketing campaigns and optimization. Even the legendary cold drink company, Coca-cola, uses predictive analytics, collecting data from social platforms, vending machines, and customer surveys, to improve their targeted marketing.
Customer segmentation
It’s easy to segment customers based on simple variables like region, age, etc..
But if you have to perform advanced segmentation beyond demographics, you will need predictive analytics ML models. For example, segmenting customers who are likely to buy a product or prefer a feature, or those who might churn, and the like. Such categories require advanced data manipulation, which predictive analytics can do for you and produce customer segments based on prediction basis.
This way, you could narrow down campaigns further, target with a more appropriate message, reach them out on channels they are available, and receive expected impressions everywhere.
Based on the results of campaigns conducted on each segment, you could further optimize the model and receive accurate results next time.
Predictive analytics is your go-to if you want more granular segments for effective targeting in marketing and sales.
Customer churn prediction
Predictive analytics models can find out accurately customers who might leave your business. Churn patterns can be different for each business and industry. With a model customized with relevant churn features and predictive variables, you could generate customers who might discontinue your product or service. Some predictive variables could be ‘customers with high customer support tickets’, ‘customers who give low ratings’, ‘customers who delay renewals and subscriptions’. Or anything that means a negative move from customers’ side.
These churn models in marketing assign churn predictor scores to each customer and segment them into categories like low-risk, medium-risk, and high-risk. Depending on the category, you could prioritize retention campaigns and secure customers back. Combining churn prediction results with product analysis, marketers could perform root cause analysis, fixing churn not just temporarily, but for a long-term.
Personalized campaigns
83% of customers are okay with data sharing, if it’s for a more personalized experience. Marketers are happy about this, but are equally confused when it comes to hyper-personalization. Predictive analytics in marketing to the rescue again. It can help you understand customers, their behaviors, preferences, needs, and the whole picture beyond it. Based on their past purchase and behavioral experience data, you could understand what type of message they respond to.
Predictive analytics also help with dynamic and micro segmentation, where you segment customers based on their behaviors - discount seekers, buyers, and churners. Each segment receives alerts and messages personalized to their preferences and buying patterns. Hyper personalization is more than this—dynamic discounts, exclusive deals, and loyalty programs based on response predictions. All of which exhibit the right level of personalization, where the user feels being valued and not creepy.
This predictive analytics use case can help specifically with eCommerce marketing, where pop-ups, push notifications, and email marketing go out every minute.
Recommendation systems
A recommender system can work great in digital interfaces, eCommerce platforms, and product interface, sharing information or products users might be interested in. It’s like how Amazon suggests products you are likely to buy next.
Such recommendations are mostly dynamic and change based on user behavior, actions, clicks, purchases, etc.. Aggregating and processing this data real-time, predictive analytics could offer personalized and contextual recommendations. User interactions and feedback can further enhance the model's outcome.
Recommendation systems are effective for eCommerce platforms, social platforms, streaming applications, and similar systems.
Predictive analytics in marketing can help with the following.
Suggesting content not only based on user interactions but also users’ connections.
Recommending what people like them are interested in.
Optimizing advertising content and allowing marketers to do A/B testing.
Know customers’ behavior and suggest to them exactly what they might be looking for.
Have a scalable model that grows with your data and share reliable, accurate predictive insights required for personalized recommendations.
Lead scoring
Predictive analytics are effective for lead scoring, which determines how likely a lead can convert into a customer. Traditionally, lead scoring is a complex method, involving various factors, from demographics to behavior analysis to firmographics (for B2B). Also, lead data can be traced from various sources - website, customer inquiries, CRM, etc., making it impossible to have an accurate lead score.
However, predictive analytics can overcome these challenges, integrating marketing and CRM data and standardizing lead scores despite dynamic conditions.
Here’s how you could come up with accurate lead scores in marketing using predictive analytics.
Finding out behavioral triggers of customers that determine lead buying intentions.
Building a predictive model with relevant features to predict, score, and segment leads.
Running targeted campaigns customized for each lead segment to bring them down the funnel.
Improve campaign ROI and conversion rates, which help both marketing and sales team work in unison and achieve respective goals.
Demand forecasting
Predictive analytics also help with demand forecasting, a specific application of predictive analytics used to predict future demand of products/services. While predictive analytics is more of a broad term, demand forecasting is a specific prediction act of changing demands between a specific duration of time.
Demand forecasting can help marketers know what’s likely to be sold more in a few days, hence promoting that through their activities.
Here’s how predictive analytics play a role in demand forecasting for marketing.
Run a time-series or advanced forecasting model, connecting an array of data sources like sales, historical campaign results, external factors, etc.
Handle complex demand predictions, including seasonality, trends, and non-linear relationships between demand variables.
Perform simulations and what-if analysis to run various scenarios and how they impact demand in real-time, so that marketers can optimize campaigns better and achieve high ROI.
Let the model improve with time and data to share predictions that are more accurate.
How to implement predictive analytics in your marketing team?
Implementing predictive analytics for marketing operations requires you to set up objectives, build models, facilitate data management, and analyze results. Here’s the step-by-step to perform predictive analytics for marketing.
Set the objectives
It starts with identifying requirements and use cases within your marketing team. Speak with marketers to identify their problems, be it with lead scoring, personalization, customer retention, or ROI improvement. This leads to setting measurable goals - improve our ad campaign success rate by 20% or reduce churn by 10%.
Get the relevant data
Depending on your goal, required data will vary too. Gather required data to meet your marketing prediction goals. This includes identifying, integrating, processing, and data from CRM, website, marketing automation systems, customer data, or anything required. With the help of data cleansing and transformation tools like ETL, process the data, set up pipelines for stream or batch processing, and make the data standardized and ready for analysis.
Perform feature engineering, which requires turning variables from current data into more new variables, apt for predictive modeling and analytics.
Build a model
Before building a model, choose the right predictive analytics technique depending on your prediction goals. If you need customer segmentation for personalized marketing, you can choose clustering algorithms like k-means clustering. If you want to run recommendation systems, you can select techniques like collaborative filtering, content-based filtering, etc.
If your data share non-linear relationships, then you could go for advanced algorithms like gradient boosting or ensemble models for more prediction accuracy.
Then comes the model building, training, validation, and testing. You will require data scientists and analysts to perform tasks listed in the last and current section.
Data scientists will select a suitable, scalable model, divide data into training and testing sets, probably in a 3:7 ratio. The scientists will train the model with the test data and help the model learn explicit and hidden relationships between chosen variables.
Using the training data, they will evaluate the model accuracy and perform the necessary adjustments.
Educate your employees
Building a model isn’t enough if no one knows how to operate it or how to incorporate its outcomes into existing workflows. Help your marketing team get familiar with predictive model outcomes and how to read, understand, and use them for marketing campaigns. Bring predictive analytics into the core of marketing, and use it for lead scoring, personalization.
Also, educate your team on how predictive models work, and whether the results are weekly, bi-weekly, or day-based.
Analyze and refine
After 3 months into deployment, analyze if the marketing team could meet the goals set during the initial process. These analyses will help data scientists tune models and fix any accuracy and bias issues that arise. Once the first predictive analytics model for marketing is successful, you could further expand into other use cases like up-selling, cross-selling, ad spend optimization, and others.
Final thoughts
‘Everyone is not your customer,’ says Seth Godin. That’s what marketing teams do, ensuring your message appears only to the right people. Yet, in a crowded market and distributed customers, each with varying behaviors and trends, it can be hard to get the message across. Predictive analytics can be a crucial tool for them to bridge the gap and become more effective in marketing activities. If your marketing team is struggling with catching the audience’s flow and is unable to meet marketing goals, predictive analytics might be the catalyst you need. With datakulture, you get tools, data professionals, and the roadmap you need to implement predictive analytics, and expected results.