Predictive analytics in healthcare
Predictive analytics in healthcare helps healthcare professionals make accurate disease diagnosis ahead, speed up drug development, and improve operational efficiency at facilities. The evolution of this life-saving analytic technique, predictive analytics healthcare use cases, real-time examples, and more details below.

Thulasi
Oct 6, 2024 |
7 mins
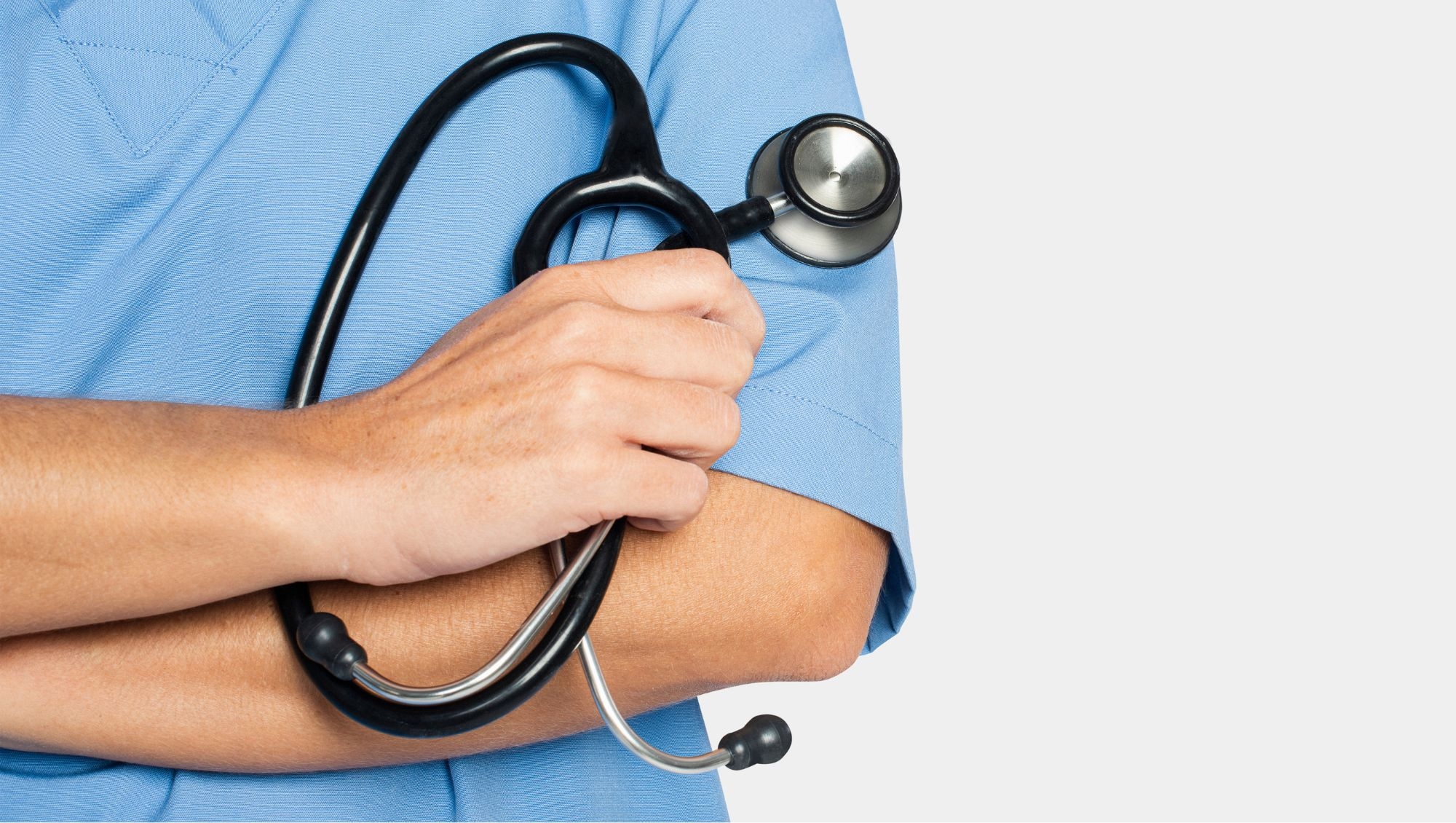
What is predictive analytics in healthcare?
Predictive analytics is a machine learning and AI-based technique that predicts future outcomes based on past and current data. Predictive analytics in healthcare uses healthcare data, makes disease predictions, identifies risk levels, shares insights into future symptoms and more, helping healthcare practitioners make informed and quick decisions.
Why is predictive analytics needed for the healthcare industry?
Predictive analytics could solve many issues that the healthcare industry struggles with - from chronic disease management to cost control measures.
The industry is home to abundant data that could be used to receive evidence-based recommendations for diagnosis, treatment, and other operational objectives.
Offering personalized care and treatment to high-volume patients, considering their medical history, allergies, genetics, and other restraints into account.
Maintaining patient inflow, staffing, facilities management, scheduling, and other administrative tasks with ease across multiple branches.
Reducing costs by preventing unnecessary testing or re-treatments by reducing the chances of complications, unnecessary procedures, re-hospitalization, etc.
Example of predictive analytics in healthcare
Healthcare institutions using predictive models for early diagnosis in the case of acute diseases like cancer. These diseases spread fast, yet are difficult to identify ahead. Predictive analytics analyze millions of healthcare datasets, symptom progressions, trial results, and treatment outcomes to identify cancer cells accurately, before a medical practitioner could notice.
Predictive analytics examples in Healthcare
Reducing hospital readmission
Predictive models help healthcare providers take proactive measures like post-discharge care, remote monitoring of vitals, personalized diet plans that modify based on health conditions, follow-up admissions, etc.
Data analysis: through continuous analysis of data like treatment details, and vitals monitoring, predictive models look for risk signs and patterns, which can get the releasing patient sick again.
On-time interventions: based on above analysis, the model can suggest insights on medication and care recommendations, even after the patient is discharged.
Remote monitoring: vital monitoring through IoT and wearables that enable healthcare technicians to stay connected with patients remotely.
Model improvement: predictive models can refine its results and make readmission prevention suggestions more accurate.
Through personalized care and timely interventions, medical practitioners can improve patient outcomes while reducing treatment costs and possible readmissions.
Predicting diseases for patients
One of the best AI use cases for healthcare is disease diagnosis. It involves the following processes.
Collecting records like EHR, medical imaging, patient details and demographics, lab results, and other environmental and external factors.
Model learns from data of patients with known outcomes and learns to connect and interpret relationships between multiple variables. Example: connecting past or genetic medical conditions with the development of a current symptom.
Anomaly detection like abnormal growth of cells through CT scans, MRI documents, etc, that could be easily misunderstood for something else.
Predicting onset of diseases based on calculated risk factors that allow early interventions. For example, early detection and treatment of diseases like Alzheimer's through genetic predispositions.
Improved clinical decision-making and accurate classification of patients into different risk categories, so doctors could provide prioritized intensive care treatment to the high-risk ones.
One good example for disease diagnosis using predictive analytics would be machine learning models predicting type 2 diabetes. It does that by analyzing BMI, real-time glucose changes in the bloodstream, along with other factors like family history.
Managing insurance claims in a better way
Insurance fraud is steadily growing with fraudulent insurance claims, estimated to be 10% out of all submissions, leading to insurance companies and healthcare agencies losing money. Predictive analytics could help here with the following.
Following suspicious patterns in claim submissions and identifying duplicate, fake, and fraudulent claims, so insurers could make proper investigations.
Quicken claim processing and segregate claims that could be auto-approved, requires additional documents, claims involving high-risk patients, etc.
Improving customer experience through quick processing of claims and thorough decision-making.
Can help insurers make dynamic adjustments to their policies and premiums through customer risk assessments.
Can stay compliant with existing regulations for the insurance industry and address any non-compliance issues ahead.
For example, a vehicle insurance company could set up auto insurance approvals using predictive analytics, analyzing driving behavior, accident records, past claims, etc.
Overall, predictive analytics could help insurers make better decisions and save millions in cost wastage that happens by approving fraudulent claims. Also, the triage and processing teams could function with more efficiency, processing increasing volumes of claims in less time.
Personalized treatments
Most chronic diseases management require personalized approach as every patient condition and response type is different.
Predictive analytics could help here, making the process effortless for healthcare professionals with improving prediction accuracy.
These AI models are quite advanced and can offer tailored suggestions based on analyzed data. For example, using systems like IBM Watson Health, personalized cancer treatment for cancer patients could be offered by analyzing research papers, patient data, and clinical trials. Here is how predictive analytics in healthcare work for personalized treatments.
Comprehensive data analysis including genetic data, lifestyle factors, medical history, and other patient specific information.
Predicting drug and dosage, depending on current conditions and how likely the patient might respond during and after the medication. This could be an effective use case for chemotherapy treatments to provide the perfect dosage to reduce the tumor, preventing any toxicity.
Based on data collected through wearables, adjusting treatment, dosages, and medication in real-time for quick improvements.
Predictive analytics could help with clinical trials, where healthcare workers could easily match the patient’s profile with a suitable trial, depending on the medical and genetic background.
Predictive analytics also finds its role in precision medicine too, which is more about personalizing therapies based on type or subtype of the disease, performing research to find which approach would work best for a patient.
All the above promotes a fast and effective way of treating a patient without wasting time on trials and errors.
Reducing cancellation and no-shows
Appointment cancellation and no-shows can be a burden for medical and administrative workers. Not only the schedule management but the risk profile of the patient needs to be taken into account too.
Here’s how predictive analytics can help streamline scheduling in healthcare.
Enabling dynamic and real-time adjustments to scheduling, which could auto-suggest best timings for rescheduling when customers cancel appointments.
Using predictive models to analyze peak load for different staffs, cancellation trends, patient preferences, etc., for effective load balancing. Bridging the gap between no-shows and busy back-to-back patient handling.
Personalized communication, reminders, and follow-ups to ensure that the patient or the care-taker is reminded on time.
Alert the team for a followup call, when a high-risk patient misses the schedule.
Predictive solutions could go beyond sending reminders and allow healthcare providers to uncover reasons and find out social determinants like income, accessibility, job, etc., which leads to the no-shows. This insight could break barriers and allow the team to provide most suitable solutions like financial assistance, transportation arrangements, etc.
Predictive maintenance
One of the major goals of healthcare institutions include protecting critical healthcare equipment, ensuring uptime when required, while increasing its lifetime. Predictive analytics could help them achieve all the above.
Continuous monitoring of data like sensor readings, temperature, vibration, etc, from equipment MRI, CT scanners, lab instruments, ventilators, HVAC systems, etc.
Detecting peak and overload performance that might lead to potential breakdown or wear and tear and alerting the concerned team.
Preventive maintenance plan & inspections for each critical equipment based on its conditions.
Failure forecasting as the maintenance model reads machine usage ratings, so early action could be taken to prevent it.
Minimizing equipment-related accidents or harm to patients or operators as it’s operated in ideal schedules.
Predictive maintenance is useful across industries to replace corrective maintenance with condition-based maintenance schedules for operational efficiency and the longevity of machinery. With a transparent maintenance schedule that’s planned ahead, staff could use and allocate these resources without clashes. Predictive maintenance in healthcare is a major use case that could prevent fatal accidents and ensure human safety as they work with high-risk, critical equipment.
Final thoughts
The role of predictive analytics in healthcare is to ensure early detection of diseases, reduce any chances of readmission, and help medical providers offer better healthcare, without being overburdened. This can be a lifesaver, as patients receive on-time treatment and get out of risky conditions, while medical practitioners get a reliable co-pilot, guiding them with every decision. Hospitals and other healthcare institutions could operate seamlessly managing everything from flexible scheduling to facility management to equipment maintenance.