Why is machine learning forecasting better than traditional methods?
Predict the future with precision and accuracy with the help of machine learning. How it works for different industries, real-life applications and use cases, benefits, and more. Read the article to know how you can have a crystal ball to forecast based on your historical data.

Jagadeesan
July 23, 2024 |
8 mins
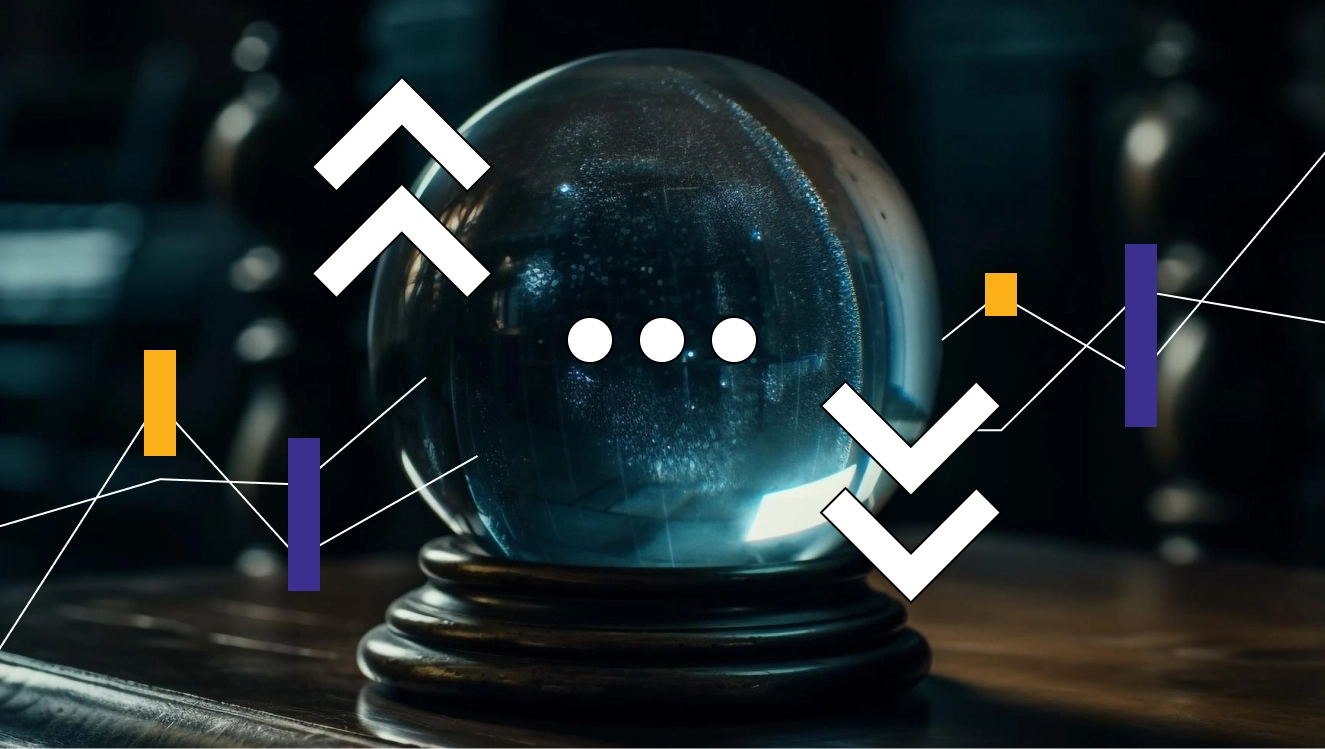
Real-time examples of business forecasting
How do huge retail chain companies know what customers are going to buy? How are meteorologists forecasting weather in advance? How do financial companies send stock pricing forecasts weeks or months ahead?
Forecasting. This is what happens in the backend through which you get a sneak peek into the future. Many businesses rely on this to be prepared, set objectives, plan and allocate resources, and brace for impact.
Here are a few examples of machine learning forecasting methods.
1. Demand or inventory forecasts in retail: ML demand forecasting models can generate accurate demands so they can maintain optimal inventory levels without stockouts or excess inventory occurrences. Imagine an eCommerce company predicting the sale of winter clothes out of the sparsely spread data from October to February which may vary during each of these months, calling for precise stock management amidst monthly fluctuations.
2. Forecasting in trading: Automatic algorithms can analyze historical stock price movements, economic indicators, and other factors to predict potential opportunities that can yield maximum returns.
3. Forecasting in healthcare: Using algorithms to predict the disease outspread in a particular region so necessary countermeasures could be taken. Eg. vaccinating people or improving hospital accommodation.
But these examples don’t mean forecasting is just for big companies. It’s for any company that wants to keep their operations in line with future requirements based on their historical trends and many other factors that possibly affect the performance. And it’s for any leader who wants to take the right step by knowing their focus areas.
Forecasting is for businesses of all sizes and it holds its place in every industry—in different ways and names.
While we are at it, it’s also important to differentiate how predictions and forecasting are two different terms. The difference between predictions and forecasting in ML terms is that prediction is estimating an unknown value using known data, whereas forecasting is about estimating the future based on historical data. Prediction isn't necessarily about time, but forecasting is always about finding what happens in the future. Prediction is more of a broad term with varied scopes and solves both classification and regression problems.
What are the forecasting techniques in machine learning?
ML forecasting uses advanced techniques like random forest, generalized regression neural networks, artificial neural networks, classification and regression trees, etc.
Depending on the use case, data availability, accuracy requirements, one or a combination of the following machine learning forecasting methods is used.
Time series methods like exponential smoothing, vector autoregression, etc.
ML techniques like decision trees, random forests, k-nearest neighbors, etc.
Advanced techniques like recurrent neural networks, gated recurrent units, prophet, autoencoders, etc.
Hybrid and ensemble models for improved accuracy.
Statistical methods or ML-based forecasting - Which method is better for you?
Statistical methods have been in use for a long time which uses techniques like moving averages, exponential smoothing, ARIMA, and ARIMAX. They are best if you have limited data and countable variables. For such univariate time series data sets, the accuracy will be reasonable and the model will be explainable.
On the other hand, ML-based forecasting shares reliable, accurate forecasting reports, considering multiple external variables that affect the outcome.
Why use machine learning for forecasting?
Choosing the model or an ensemble depends on your data and the use case. But, modern businesses prefer machine learning models for the following reasons.
ML models can easily handle multivariate data sets and learn with time as they are fed with new data. They also come with the ability to ensemble two or more different models for improved performance and accuracy.
Get accurate predictions and gain control of the situation despite numerous uncertainties.
ML models are scalable and are suitable for growing businesses with an increased number of products.
If you want a real-life example of how ML-based models can improve forecasting accuracy, then check out our case study - loan sales forecasting using ML for an NBFC company. We helped them predict sales with improved accuracy and, in addition, receive insights about business and operational factors that impact sales — with the help of an ensemble machine-learning model.
Statistical methods or ML-based forecasting - Basic differences
Points of comparison | Statistical forecasting methods | ML-based forecasting methods |
Scalability | Statistical methods aren’t for large datasets. It would require way too much effort and the accuracy levels may decline. | ML-based forecasting methods are perfect for scalability. Imagine a business with a huge range of products, expanding through and beyond its target region. ML-based forecasting would be perfect for this case. Matter of factly, the model will enhance itself as it’s getting trained on new data. You can introduce any new variable and the model will still continue to generate accurate forecasts. |
Explainability | Traditional methods are rather more explainable. You can interpret and explain the forecasting outcome. | Depending on the model complexities, explainability may vary. The more complex a model is, the less explainable the decision process becomes. |
Accuracy | You can expect a reasonable accuracy if the amount of data is limited with a lesser number of variables. | ML forecasting models have always shown higher accuracy levels compared to traditional models, even if they are exposed to different datasets. |
Forecasting factors it can consider | The prediction looks best with univariate datasets. | ML models let you add many internal and external factors, like competition, economic conditions, social media trends, etc. |
Promptness | Their promptness is inversely proportional to the amount of complexities in the process and the size of the dataset. | ML models are faster in performance and don’t lag no matter the amount of data you ask them to compute. |
Ability to explain complex, underlying patterns | Statistical models cannot understand or explain sudden spikes or plunges in the data. It also cannot explain what factor drives the forecast outcome and how. | ML-based models can do both; pick any minute trends and patterns underlying, explain how driving factors impact the outcome. They can also adapt to any new changes in the data. |
Advantages of machine learning-based forecasting
There is a reason why you should lean on ML-based forecasting methods over statistical models.
1 - They are highly accurate. With the right prediction comes steady and healthy progress of your business. It can also adapt if you expose them to more data and still exhibit the same level of performance and accuracy (low volatility). This is the exact opposite of traditional forecasting models where accuracy declines with time and different data.
2 - You can use a huge amount of data and a wide range of factors and still get accurate forecasting results and inferences.
3 - ML forecasting methods require the least amount of human intervention to perform all those heavy computations.
4 - Machine learning forecasting models aren’t just for linear datasets. They can deal with datasets that share both linear and non-linear connections and identify any complex relationships, trends, and underlying patterns.
5 - These modern models take into account various external factors like weather info, competitor details, social media trends, customer tendencies, and lots like this.
Forecasting in different industries
Every industry has its own set of requirements to predict ahead. See how these industries can utilize forecasting and how machine learning can play a part there.
Forecasting in retail
If there is one industry that couldn’t do without forecasting at all, then it’s retail. They are also forerunners in using forecasting methods to stock up on what might sell. Only the complexities have increased with time, given the additional factors influencing sales—season and weather, festivity, pricing, internet-driven trends, and more.
Think of an apparel company selling clothing and shoes and use demand forecasting based on machine learning algorithms. They discover a surge in a specific cardigan brand, recently popularized by a pop singer. By acting promptly, they can meet customers’ demands, exceed sales targets, and boost revenue—ahead of competitors.
If this is for one product, imagine how many products a retail business sells daily. Add to that their branches, locations, and target audiences, and the shifting market conditions, stressing the importance of machine learning.
What type of ML based forecasting retail companies must perform?
Demand forecasting
Take a look at this study by the IHL group. It points out that overstocking has caused $562 billion loss and understocking has caused $ 1.2 trillion for the fashion industry. Not only fashion, every mortar and brick store faces similar challenges—even more so for stores with perishable goods.
This is why retail companies must be data and demand-driven. So that they can plan their stock intake, sale campaigns, discounting, staffing allocation, and even the arrangement of these stocks on shelves.
Given the mammoth amount of data and independent variables, machine learning models will function the best here, sending weekly, monthly, and quarterly forecasting for each product, category, store, and location, considering as many variables as you select.
Real-life use case of demand forecasting:
H&M is using ML for demand prediction. With their team of data scientists, they are collecting data—lots and lots of them—on people’s fashion preferences, what they are looking for, what they like, what’s trending, and more. This is what their demand prediction looks like which powers their operations—stocking the right outfits across the right stores in the right quantities.
Forecasting in financial services
Industries like BFSI are highly volatile and are dependent on multiple external factors. Accurate forecasting is crucial for them as they navigate through investment risks, find ways to increase profits, and sell the most required products.
Following are how financial industries use forecasting to drive their business value.
Risk forecasting
Banking, finance, and insurance industries are more prone to risks and fraud—all kinds of them. It can be through external factors, employees, credit fraud, market fluctuations, operational issues, and cybersecurity. And if it’s not forecasted, chances of incurring losses are high—financial, reputational, or both.
With an increasing number of risks, you can’t imagine possible risk scenarios and be prepared with mitigation strategies.
Rather, you can perform risk forecasting, identify potential risks, and act on them on time.
For years, many finance companies have been relying on statistical risk forecasting models and pro forma statements.
However, these may not be enough as risks can be due to multiple reasons. This is where ML forecasting models help. Many banks and NFBC companies already have risk forecasting systems in place that tell in advance financial, strategic, operational, and reputational risks they may face.
Sales forecasting
This one is common for almost every industry yet crucial for the finance, banking, and insurance sectors. This is because of the cut-throat competition the industry faces, the need to have the cash flow planned ahead, the varying economic trends, and the rigid compliance requirements.
Time series analysis is also used where previous historical sales data along with seasonal trends and spikes get analyzed to predict future sales.
It not only offers them the predicted figures but also various features and factors that might impact the sales.
Forecasting in supply chain and manufacturing
Supply chain and manufacturing industries constantly have to keep up with the demand and combat wastage. As the whole industry is driven through the collaborative efforts of different teams, forecasting figures can be that balancing yardstick, bringing everything in line together, and helping them make effective decisions.
With umpteen unpredictable seasonal trends, machine learning forecasting methods are proven more accurate here than traditional models.
Demand forecasting
In manufacturing and supply chain, demand forecasting is predicting future sales and customer demand to fuel the manufacturing process. So they go in sync with market conditions, customer demands, seasonal trends, and sudden surges they notice in their sales cycle.
Traditionally, methods like moving average, exponential smoothing, and the Croston method are used to predict this demand.
But the best way ahead for heavy, multivariate data sets is to use ML algorithms like time series analysis.
With this advanced setup, you can get a demand forecast as descriptive as possible. Ex. how the demand for a particular product goes down with the chances of rain or snow.
Inventory forecasting
Planning the inventory to keep up with fluctuating demands is a huge task. With demands and manufacturing varying every day, inventory management can go for a toss, affecting supply.
Forecasting is how you can optimize this end-to-end—from manufacturing to distribution without facing loss and wastage.
Exactly what Walmart’s smart forecasting team is doing every week—predicting what product needs to be moved to stored and in what quantities—all with high-level precision. Imagine doing this with traditional methods for a mortar and brick store with 120k+ shelved products. Math ain’t mathing, right?
Forecasting in SaaS
The SaaS industry is constantly expanding and is expected to grow by 19.7% between 2013 to 2020. This not only highlights the growing demand in the market but the underlying cut-throat competition as well. To be the customers’ choice, SaaS companies must have to break a few shackles, ditch conventional methods, and rely more on data. Be it their customers’ reviews, market research, or forecasting data.
Following are a few forecasting methods for the SaaS industry.
Customer churn forecasting
Retaining a customer >>> Bringing in a new customer. Customer retention is and will be a major priority for SaaS companies. But how can a company know which customer is likely to leave and which one is loyal? Churn forecasting can help here. This is not just for SaaS but any industry that wants to prioritize focusing on existing customers. To name a few, banking and insurance, media and entertainment, telecom, etc.
The churn forecasting results will help the business see who is likely to leave; so they can focus on them, fix their concerns, share goodwill discounts, etc.
Revenue forecasting
Revenue is a major growth benchmark for SaaS companies. By accurately forecasting it, they can align their sales, marketing, and product strategies in a way. Unlike traditional linear forecasting methods, you can make tuning adjustments on the data variables to see how one variable affects the end outcome, your revenue.
For example, you can see how sales activities can impact the revenue. This detailed analysis level opens doors to many explorations, allowing you to make the right moves and select the most promising options.
Forecasting in healthcare
With gazillions of data on disease history, patient information, treatments, and progress, healthcare could use machine learning to solve a few critical problems. By feeding them to forecasting models, we can see unexplainable patterns behind the spread of a disease, patients’ survival rate, symptom development, drug absorption and reaction rate, etc.
These insights can help healthcare professionals offer proactive treatment and take the right preventive measures—all of which are literally life-saving.
For instance, check out this study conducted with the electronic health record data of 5 hospitals to identify the mortality rate of COVID-19-affected patients to predict the development of symptoms on consecutive days. All of these could have helped the institutions identify at-risk patients, predict any signs of underlying diseases before the body starts showing symptoms, take the necessary steps, and improve their chances of survival.
A real-time case here. Mayo Clinic is gathering and analyzing patients’ data with the help of ML forecasting models to prevent, treat, and find novel healthcare solutions.
Other than this, forecasting can be also implemented to analyze hospital occupancy, healthcare workers and equipment availability, the emergence of epidemic situations, etc.
Final thoughts
“We look at the present through a rear-view mirror. We march backward into the future.” - Marshall McLuhan
This quote very well fits for forecasting if you equate it to the rear-view mirror. You see the past and present, and connect the dots to know where you are heading.
As a leader, you might make countless decisions every day—significant ones, not-so-important ones, and trivial ones. You use your intuition and judgment along with collective opinions from people around you, analyzing multiple factors. But this isn’t enough in a world driven and dominated by uncertainties. An accurate forecasting report from a strong model can help here, elongating your cone of uncertainty as HBR calls it, taking into account the outlier factors you missed, and sharing trustworthy insights and inferences.
Machine-driven forecasting + human intelligence - Core of effective decision-making
That’s how you become data-driven and make better decisions seamlessly and effortlessly.
However, building a scalable and customized forecasting model requires effort. Your current data has to be prepared. The right model must be chosen, built, trained, deployed, and monitored. All of this requires a skilled team of data scientists and analysts.
With expertise in building forecasting models with the highest accuracy, datakulture can meet your needs and ensure on-time implementation.