Data governance guide
The only data governance guide you need, covering data governance definition, benefits, challenges, and best practices. Our data experts have shared their two cents on everything from meeting compliance requirements to securing privacy to metadata management. Learn how to set up robust data governance principles to manage your organization’s most crucial asset, data throughout its lifecycle.

Suresh
Dec 19, 2024 |
15 mins
Key takeaways from the guide
1. Data governance is not just a set of principles to secure your data from IT, security, and non-compliance issues. It also helps you unlock the full value of the data, serving it to the right, when needed.
2. Many organizations face challenges with data governance programs due to siloed data, lack of roles and responsibilities, shadow IT, and low awareness among employees.
3. If you begin to implement or renew data governance, start it small with a focused project, measure effectiveness, and expand across the organization.
4. Data governance has 4 important pillars - data quality, data security and compliance, data stewardship, and data lifecycle management.
5. Data governance requires continuous effort and improvement. Review, regulate, and refine policies and processes to suit changing landscapes.
What is data governance?
The growth of business data is rampant. Businesses no longer store it for the sake of storing—rather, they invest in analytics, AI, and decision intelligence. It’s safe to say that data is becoming the foundation that powers organizational decisions. Many big questions arise here - who gets access to this data, how is this managed, is it safe from breaches, are we compliant with legal regulations, and all that.
This is where data governance plays a role. Data governance is the blueprint to an organization to moderate data management, ensure safe and effective use of data, and keep risks away. Like pulling the brake on a snowball rolling downhill, governance principles control the ever-growing and widely distributed data
Major aspects of data governance
If you want to understand the concept of data governance, going over data governance components is paramount.
Data quality denotes the completeness, quality, and accuracy of data, and how reliable it is for decision-making.
Data security is needed to protect sensitive data from unauthorized users inside and outside the organization.
Data privacy is how well the organization protects personal information and complies with regulations like HIPAA, CGPA, GDPR, and the like.
Data compliance is whether the organization adheres to data storage and usage policies mandated by both regulatory boards and internal stewards.
Metadata management describes the organization’s adeptness in data discoverability and understanding.
Data consistency defines if data is consistent and the same throughout the organization.
Benefits of data governance
Some benefits of setting up data governance in your organization includes:
Better decision making
Trust-worthy and high-quality data means accurate decisions that work. Data governance allows organizations to gain trust in their data and reduce decision-making risks and related cost wastage. Also, data governance facilitates real-time data availability, making it possible to make swift decisions on time.
Ensure security, compliance and privacy
Not adhering to security and compliance requirements could lead to hefty fines. Data governance prevents this from happening, ensuring that the company meets industry-specific and global standards. The company can be relieved and stress-free whenever there are audit trails, leaving with clean records and documentation.
Single version of truth
Having siloed data is often a reason for data security and inconsistency issues. Data governance applies to the entire data assets, allowing you to have a unified governance for all business data. There is increased accountability and transparency within the organization, and the architecture becomes more of a single source of truth.
AI initiatives
The success of data and AI initiatives stems from clean, well-governed data. Imagine that you find a data science or AI use case to improve a business bottom line. You will need clean and accurate data to train and run algorithms, or else will face bias, errors, and inconsistencies with insights. Underlines why data governance is crucial for AI and data science use cases.
Reduced costs
Data quality and inaccuracy issues can be too expensive to fix. On top of that, you will be paying high storage costs, when there is duplicate data without clear metadata management. Data governance minimizes these operating costs, with optimized storage, fewer reworks, and proper compliance adherence, aligning data use cases with business goals.
Challenges of data governance
Many organizations face the following challenges with data governance.
1. Siloed data
Fragmented data sources and data sources scattered across departments are the major challenges of data governance. Unless the company establishes a single source of truth and cross-functional collaboration, connecting disparate data points, data management is gonna be difficult.
2. No clear roles and responsibilities
Most companies think that it’s the role of the IT department to manage data. There is a lot of ambiguity about who owns data, who manages it, who is the steward, and who is the consumer. All these roles are often overlapped, leading to mismanagement, no accountability, and even security risks.
3. Data ecosystems are getting more complex
So many tools, platforms, cloud and on-premise storage sources, and analytics systems. It doesn’t end there—these platforms have structured, unstructured, and semi-structured data, scattered across everywhere.
The data teams are overwhelmed, as they should meet end-user requirements while ensuring tight security and compliance.
These challenges multiply if the organization has a global presence; they might have to think about protecting their data and ensuring changing compliance requirements that vary based on locations.
4. Accessibility and security must go hand in hand
The right user must be able to access the right information at the right time; unauthorized users shouldn’t stand a chance. This is a task, given the complexity of users, nuances of roles and responsibilities, and the growing volumes of data and analytics. The organization and its data team should draw the line, striking a balance between data utilization and security compromises.
5. Metadata is the heart of the matter
Metadata is the data about your data. Think of this as a catalog that a library manager uses to identify, mark, and track books. But, data teams find it hard to manage this, hence it either goes undocumented or poorly managed. It feels like they need metadata to understand their documented metadata, where the whole point of having this is to simplify discovery and understanding.
6. Shadow IT
The use of unauthorized devices and software is shadow IT. In most cases, IT and data teams cannot see this, not only the tech stack, but its data as well. These unregulated data sources could become a huge threat to an organization's cybersecurity, governance, and reputation.
7. Awareness among employees
Data culture can truly flourish when employees know and understand data governance policies and repercussions for not following them. When leaders or employees resist governance initiatives, it could become a serious issue to data adoption and data-led growth.
Data governance best practices
With data governance challenges like data silos, awareness issues, poor accessibility, and shadow IT, does businesses stand a chance to set it up right? Yes. By following data governance best practices, you could avoid some of the above challenges, aligning tech, people, and processes.
1. Start small and scale it after
We always insist on starting small, testing how it works, and implementing the learnings for all data experiments. That applies to data governance too. You could start with setting up a micro data governance model with its essential components and pillars - data quality, stewardship, protection, and compliance.
Focus on one issue at a time that could bring immediate impact - improving data quality, ensuring GDPR compliance, etc.
Set measurable goals
Set up a small team composed of one data owner, data stewards, and IT/Tech teams.
Collect feedback and refine the process.
2. Have executive sponsor
It’s highly advisable to have an executive sponsor for data governance if you want your initiatives to succeed. An executive sponsor in governance means appointing anyone as a data advocate, especially from the c-level leadership (CEO, CTO, CDO, CFO, or business unit heads, etc.).
The responsibilities of the executive sponsor for data governance:
Aligning business objectives with data governance goals, or ensuring if it's all aligned.
Helping the data team with securing required funding, tools, or hiring people.
Helping to secure a ‘yes’ from the business heads and management.
Why is an executive sponsor needed for data governance? Many data teams find it difficult to get the required support from all stakeholders. Having a senior person can bridge the gap and even out any differences between them all. Also, in growing and large enterprises, there will be a considerable resistance to change, which might hinder data governance initiatives. An executive sponsor is necessary to convince them all and encourage accountability.
Responsibilities of an executive sponsor of data governance
Defining and supporting the strategic vision of the governance committee.
Have a mindset to employ data culture and promote adoption across all levels
Stay in touch with business heads, partners, and top executives and advocate data
3. Centralized data
Centralizing organizational data favors data management and governance a lot, as it takes care of consistency, accuracy, and availability. Does that mean you should build a single source of truth? Yes. In a way. Integrating all data sources from every nook and corner helps your business in the following ways.
No more data silos. No separate versions of data that leads to duplication. Example: the same customer data is present in both sales and customer support.
Easier to zero in on quality issues like inconsistency, duplication, and missing values.
Can devise data governance policies for the entire organization and apply them. That applies to data security as well. Can enforce RBAC and other data security principles to allow only authorized users to access data.
Easier to follow the metadata approach. Users can understand data, where it comes from, who holds ownership, purpose, and everything.
Auditing and compliance are more streamlined and straightforward, as everything lies in one place.
Many companies suggest centralized data management for less IT overhead and storage costs. So, SSOT is not only beneficial for data governance but also for saving costs.
4. Build use cases
Building use cases is one way to gain stakeholder buy-in and guide the implementation team on data governance. It involves choosing any one business objective that could impact data governance results and proceeding to bring it to life. One good use case example could be cleaning up master data and performing deduplication to improve email campaign results. To implement this use case, you will define the objective and select the data governance focus - which is improving data quality and reducing duplicates. You will select the roles involved and make them as data stewards and data owners. After three months, you will measure its effects - how much email campaign results have improved.
Building use cases is the way to bring step-by-step improvement that enhances business processes as well as governance measures.
5. Select the right metrics
How to measure successfully the success of data governance? With the right metrics—actionable, measurable, and aligned with business goals. Not every company needs the same set of metrics; cookie cutter approach might not work here. That’s why you need to define your metrics carefully.
For example, a finance company might want to focus on compliance with GDPR and SOC. However, a retail company would prioritize having high-quality data.
Here are some of the common data governance pillars - data quality, data consistency, data security, compliance, data usage, and metadata management. While all the pillars are essential, you could change the order of priority.
Do’s and don’ts while measuring data governance
No need to chase vanity metrics. Select metrics that could provide insights and lead to improvement. Example: how much time it takes to resolve data quality issues.
Select quantifiable metrics. For example, data accuracy percentage. Time it takes to grant or revoke accesses.
Use dashboards and automated checks for constant vigilance.
Have metrics for all stakeholders - IT teams, business users, and compliance teams. How quick the business team is able to find the required data. Or how fast the IT team can fix tech issues.
Focus on continuous improvement - a metric may not be relevant for years. Hence, refine it periodically.
Major data governance metrics to measure its success
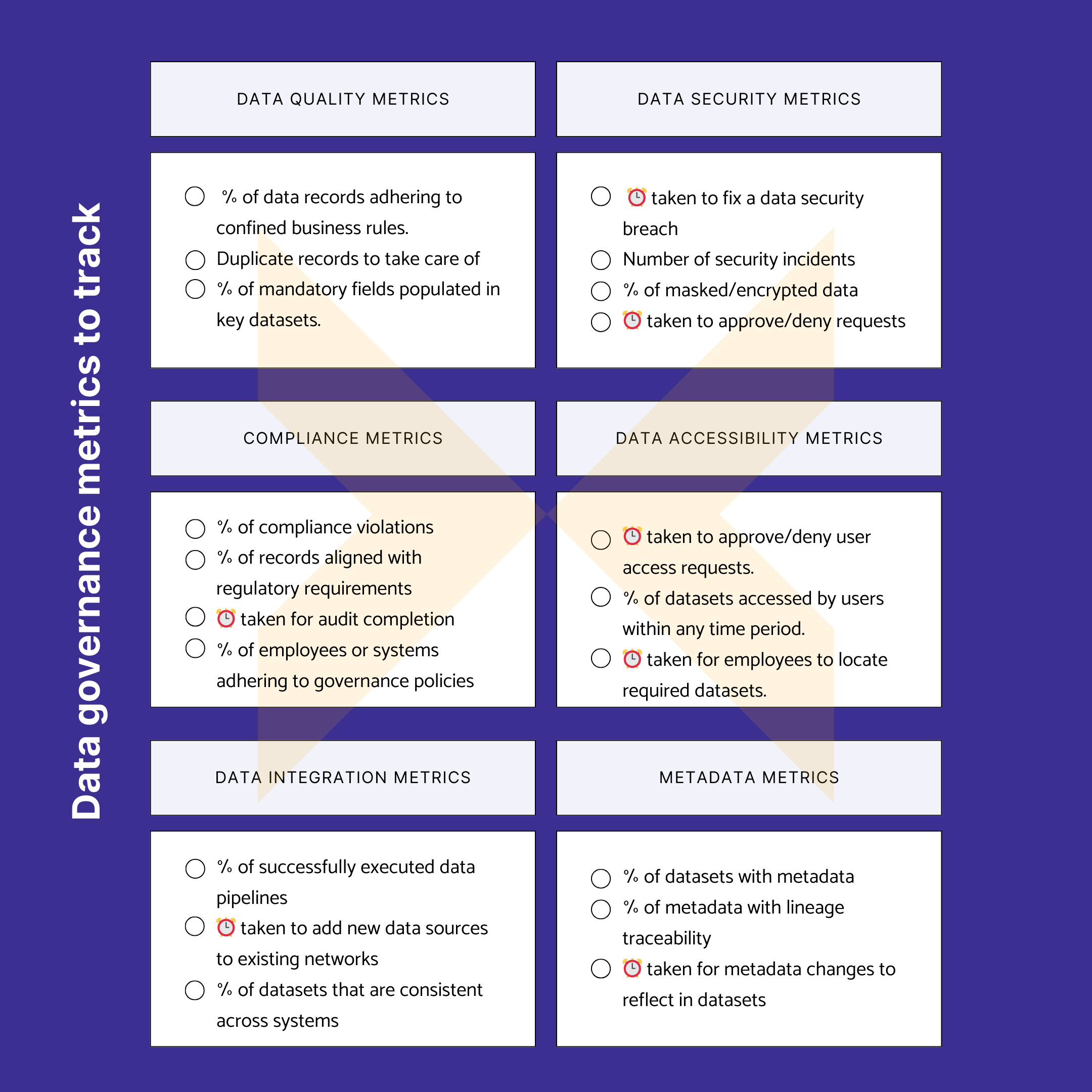
6. Raise awareness among employees of all levels
All the above measures will not pan out the way it should, if it doesn’t happen with employees’ co-operation. Employees must have a general level of understanding and awareness about data issues, compliance, and the risk of not following them. So, whatever measures you follow, ensure that it has room for employee awareness training and camps.
Many companies build data governance awareness programs for employees. You could do the following, if you don’t have time for a full-fledged training and planning.
Sending out monthly newsletters that talk about tips, success stories, and any other updates.
Partnering with third-party education providers to assign periodic courses for employees to participate in.
Any question they have related to data governance, they should have a data steward to talk to.
Emphasizing safe data access practices in every way possible. Example: Townhall or leadership meetings.
Pillars of data governance
There are five to six data governance pillars, also known as data governance components. To set forth data governance, you will require all these components to be in effective order.
Data quality
Data quality is a crucial pillar of data governance. It is what makes your data accurate, consistent, and reliable for utilization. Data quality is important because poor data quality leads to incorrect insights, operational inefficiencies, decision-making errors, and cost wastage. This is why data governance practices must focus on keeping the data clean and high quality, doing the following:
Use data validation rules to detect quality errors
Set up data profiling tools to fix data quality errors and measure the quality of datasets.
Remove duplicates and inconsistencies; employ frequent monitoring.
Data stewardship
Data stewardship is another cornerstone of data governance, which means assigning guardians to manage data assets and ensure integrity. Setting up stewards can ensure that data is managed properly throughout its lifecycle.
Here’s what you need to do while taking care of data stewardship.
Assign data owners and data stewards across the company, owners to ensure data accuracy and stewards to see to the day-to-day quality checks and governance.
Everyone’s roles and responsibilities should be clearly highlighted.
Provide training to the selected owners and stewards on current data governance practices.
Data protection & compliance
Data protection is securing data assets from unauthorized access, security breaches, and other unlawful occurrences. Securing the company data is the major pillar of data governance, given the alarming rise in cyber incidents and breaches within and outside organization.
Here are the things you could do to ensure data security, privacy, and protection.
Set up RBAC (Role-based access control), which establishes a balance between accessibility and security, preventing unauthorized entries.
Using advanced login methods like multi-factor authentication (MFA), so that it would require more than a password to break into a system.
Going for data encryption to protect sensitive data like customer data, transaction details, etc.
Initiating frequent audits and vulnerability assessments.
Data compliance also lies at the heart of the data governance, requiring the company to adhere to these requirements. You must do the following to ensure data privacy and compliance adherence.
Implementing data anonymization and data masking to protect sensitive information.
Update privacy policy regularly to align with current industry and business requirements.
Stay abreast of current compliance changes and update governance policies accordingly.
Data retention and deletion must be in line with legal needs.
Start using automated data compliance check tools like OneTrust, Microsoft GDPR assessment, etc.
Data lifecycle management
Data lifecycle management denotes managing data from its creation to deletion, extracting its full value and securing its privacy throughout the lifecycle. Data management is a pillar or component of data governance, which is required to keep the storage costs and risks low, while keeping it relevant for its users.
There are six phases in the data life cycle - data creation, data storage, data usage, data sharing, data archival, and data deletion. So, when an organization is building data lifecycle management, it must be mindful of all these stages, as data moves through each of these. The stages of data lifecycle along with the responsibilities of the data teams to ensure proper governance are as follows.
Stages | What happens during this stage? | Data governance activities and responsibilities |
Data creation | Data is created at a source or is collected from a source | Define & ensure data standards - from naming convention to format to checking if it has all must-have fields. Assigning ownership Compliance verification, if it’s required depending on the data type. Defining metadata - data source, owner, purpose, etc. |
Data storage | Data is stored in a warehouse or a Lakehouse for further processing/analysis. | Enforce access control, encryption, and other security measures. Classification of the data using respective labels. (sensitive, public, and more). Fulfill backup and disaster management responsibilities. Define - How long the data will be relevant/can be used, to free-up storage and discard when it cannot be used. |
Data usage | Data is in the form of insights now, used for analysis, decision-making, or other use cases. | Track who accesses it, who can access it, RBAC controls periodically. Validate for data accuracy, consistency, and completeness. Data compliance check once again, to see if the processed data adheres to internal and external regulations. |
Data sharing | Data is shared within teams, across teams, or with partners, external stakeholders, or the public. | Check if the shared data meets sharing terms and restrictions. Is there any sensitive data that needs to be masked. Data movement tracking to ensure secure sharing. Documenting records for further audit needs. |
Data archival | Data is no longer serving purpose; hence archived for back-ups. | Archival timelines must meet business and industrial norms. Only those who can access the archival data can access it. Stored format must be supported, accessible, and understandable. Update metadata with archival and retrieval notes |
Data deletion | Data is no longer required; deleted from all systems, including back-ups. | Check the adherence with deletion policies - checking when and how the data should be deleted. Use data deletion tools for safe deletion, so that it must never be recovered. Double validation to see if data is completely removed without traces. |
How is data governance different from data management?
Both data governance and data management are look-alike principles, but their focus and purpose vary. Data management focuses on implementing, managing, and maintaining data systems, tools, and workflows. Whereas, data governance is more about laying the rules for how this data should be implemented, managed, and maintained. Data governance establishes policies, standards, roles, and processes to extract the best value from data and use it securely.
Data management organizes your data to make it available, usable, and scalable. Data governance ensures that the data is accurate, reliable, compliant, with a focus on ownership, accountability, and ethical usage.
What’s the scope of both concepts?
Data management is operational and tech-driven. It involves handling day-to-day processes to manage data efficiently. The scope of data governance is often policy-driven, requiring strategic inputs from compliance, IT, data, and business teams. Unlike data management, governance involves creating frameworks to drive decision-making using data.
Who takes responsibility?
Data management is handled by IT teams, data engineers, data analysts, and database admins. The data governance council, chief data officer, and data stewards handle data governance.
Data tools
Data management requires tools for the following purpose: data storage tools like Snowflake, data integration platforms, ETL/ELT pipelines like FiveTran, data processing, analytics interfaces like Power BI and Tableau.
Data governance requires tools for metadata management, compliance check, cataloging, and governance policy enforcement, which is done using governance tools like Collibra, Alation, Microsoft Purview, and Informatica.
Final thoughts
With the amount of data every organization is handling nowadays, data governance could no longer be a luxury, but a part of their day-to-day operations. Besides, there is an increasing amount of regulations that keep changing every day. To win amidst all this and maintain reputation among customers, a business requires a well-formulated data governance strategy. Not to be frightened here; formulating a strategy isn’t the hardest part, but the foundation to it all. Once you set up the right team, tools, and processes, and automate using the right tools, it will be much less of a laborious task. Since smart data management and governance are key to your business's success, start small and start it now, if you would like to drive data-driven culture and growth in your organization.