The role of predictive analytics in logistics explained
The Logistics industry deals with abundant data everyday, filled with rich business insights. How can the industry use this to predict the future and face unprecedented challenges? One way is to apply predictive analytics. Discussed further is the role of predictive analytics in supply chain and logistics.

Thulasi
Sep 4, 2024 |
9 mins
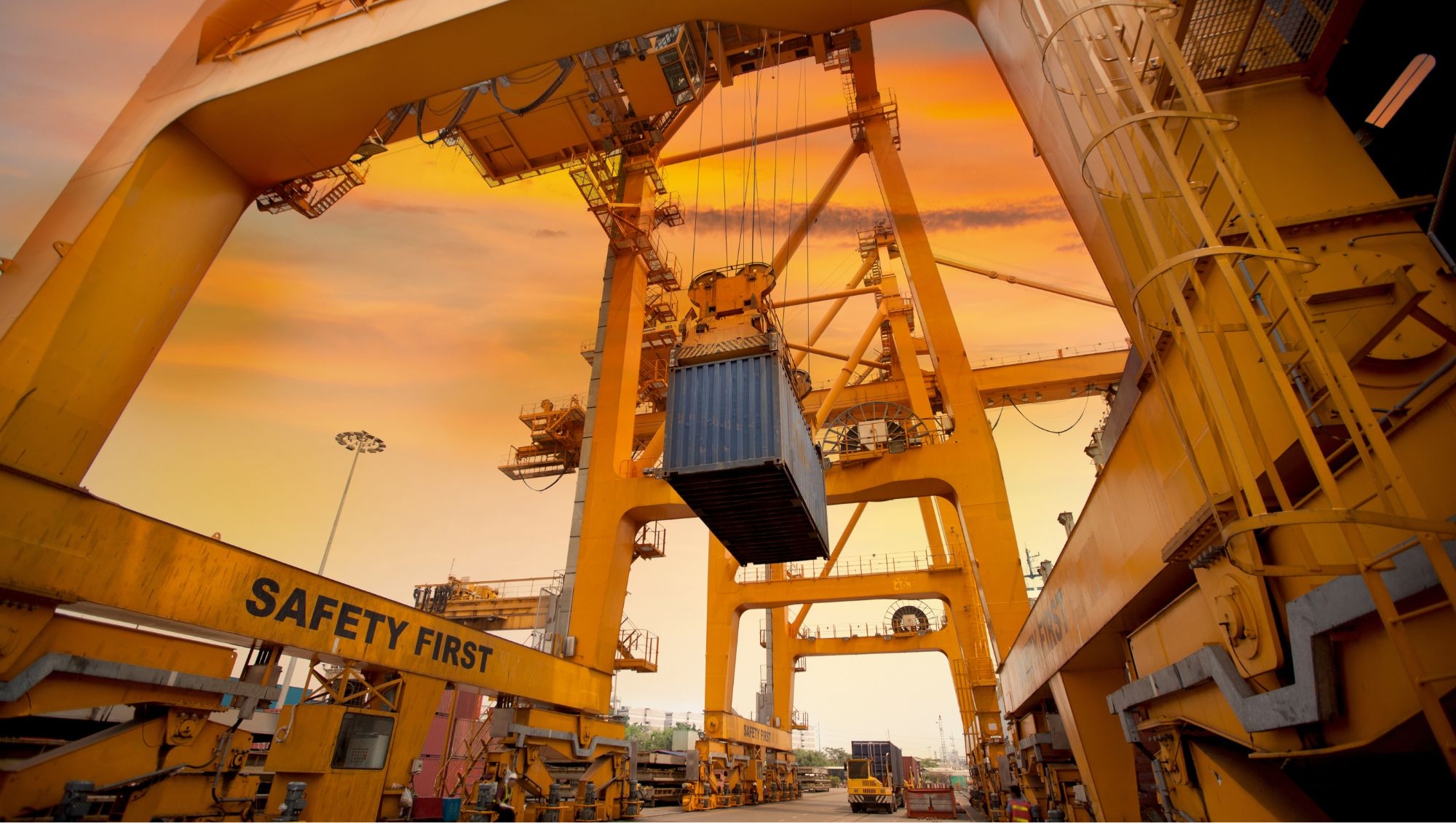
What is predictive analytics in logistics?
Predictive analytics is a type of advanced analytics that uses historical data to determine what’s going to happen in the future. It starts with data collection and involves building predictive models, performing data analysis, and visualizing final outcomes so you can understand better.
This concept works pretty well for logistics and supply chain industries, improving their operational efficiency, productivity, and reducing costs. There are many use cases of predictive analytics already in use by huge logistics companies. Route optimization, inventory and demand forecasting, and smart slotting are some of the most popular logistics use cases.
Think of all the data logistics units deal with. Data from in-bound and out-bound operations, order books, IoT devices data, and lots like this. If they could all come together, along with some external and internal factors, to predict likely events happening in the future, that’s what predictive analytics is all about.
AI-based solutions make it happen, processing huge amounts of data and drawing reliable conclusions.
For an example, consider Amazon, which does predictive analytics at scale, analyzing customer purchase data, history, buyer trends, and similar data for smart fulfillment and distribution.
Predictive analytics in logistics
Let’s discuss some popular use cases of predictive analytics in logistics and supply chain.
Inventory management
Inventory management involves maintaining the stock levels, speeding up the picking and packing process, ensuring proper labor utilization, while reducing handling and wastage costs. More expectations. Less time. And there are unpredictable external factors like changing demands, unfavorable weather, labor shortages, and the like. Ideal inventory management needs smart predictive analytics solutions that can help with the following.
Order and demand forecasting
Supplier performance analysis
Safety stock predictions
Trend analysis.
Aging stock prevention
Predictive analytics can help with the above use cases, making inventory management more efficient and streamlined.
Inventory managers get an idea of
What stocks will sell more and require refilling
How are market and economic conditions and how favorable are they for sales, so it can be aligned with goods storage.
How well are the vendors performing?
Contingency plans in case of emergencies, demand hikes
How to manage aging stocks.
And other similar pressing concerns they need to handle every day.
Route optimization
It’s time when logistics, shipping, and transportation units are looking for sustainable solutions to transfer goods. This involves reducing fuel consumption and empty miles while optimizing goods storage on containers, while meeting their timelines and delivery goals. The good news is that AI-based predictive analytics could help them meet all these goals together, considering factors like road conditions, weather, traffic, and vehicle performance. By analyzing previous days' and weeks’ traffic conditions, delivery times, and vehicle loads, route optimization applications can share optimized routes for each vehicle, covering single or multiple pickup/drop locations.
Freight managers can also use predictive analytics for route planning, so they can find the optimal timings to deliver goods. This way, drivers can deliver fast, avoiding traffic, waiting times, and bad weather.
Here’s how predictive analytics differs from manual route optimization.
Can collect real-time or near-real-time data for improved accuracy (from sensors to GPS to vehicle engine conditions).
Adapt to current route conditions and alter suggestions based on them.
Accurately merging insights about traffic and road conditions into route planning, given the high scale of operations.
Trigger real-time updates and alerts to customers and vendors that improve customer experience.
All of this could make a huge difference for large shipping, cargo, and logistics units improving their performance and reducing costs.
Near real-time monitoring
As mentioned in the above section, the combination of IoT and AI could make a huge difference for logistics industries, enabling them to monitor operations and routes real-time.
For example, IoT devices like GPS and sensors can track vehicle route, speed, load, and other related information. Whereas, AI-based predictive analytics can process it along with other data like roadblocks nearby, traffic, delivery timelines, and more and send updates to freight managers and drivers. These suggestions can be useful for navigating swiftly and delivering faster than expected timings without stress and fuss. And, it also helps with risk management, triggering alerts whenever any potential fraud or suspicious activity is detected.
Many supply chain units already perform real-time monitoring for predictive maintenance to prevent vehicle breakdown, engine failures, and potential over-performance issues. It’s also used to track fuel consumption to alert drivers when it’s overused or has to be refilled.
Resource management
A fair share of warehouse and fulfillment operations expenses is labor and resources (equipment, utilities, energy, etc) related expenses - be it air cargo, ocean freight, or highway transportation. It’s not only about expenses; it’s also about managing them stress-free and utilizing them to full extents. With predictive analytics, logistics companies can regulate their spending while maintaining utilities in good condition.
Here is how predictive analytics help with resource management.
Predictive maintenance to alert before your equipment needs rest or attention.
Forecast the demand accurately and manage the workforce based on expected business activity.
Maximizing storage and adopting smart stocking distribution with the help of predictive logistics
Align production plans and schedules with expected demand, preventing wastage of raw materials, machine operating costs, fuel, etc.
Risk mitigation in supply chain (from engaging with the right suppliers to maintaining safety stock levels to preventing resources exhaustion and breakdowns).
Demand prediction
Many logistics units keep up with the changing demands with the help of predictive analytics. It starts with analyzing historical data, identifying patterns, and deriving useful analysis of products and categories with increasing demands. While demand prediction has been performed using traditional methods for years, it cannot match the accuracy of predictive analytics that involve complex ML models like neural networks or time series analysis.
Knowing the demand accurately can help you with serving exactly what your customers want, without storing too much in the inventory and managing any supply chain disruptions with ease.
Why is it difficult to apply predictive analytics in real-life situations?
Predictive analytics comes with many impactful use cases and has a number of benefits for logistics industries. But it also comes with loads of challenges, making it difficult to approach predictive logistics operations.
Having large amounts of data
Not only logistics, many other organizations are swamped with oceans of data. Some of this might be useful, while some isn’t. While data is the prerogative for any AI or analytics projects, too much data can definitely lead to bad outcomes. Here is how high quantities of data make predictive analytics more challenging.
High infrastructure costs
Needs months to process the data and remove duplicates, inconsistent values, and other errors that might affect the output.
Overfitting risks where the predictive model is unable to generalize the data and understand the pattern behind
Unable to get meaningful insights that serve purpose and value.
So, it’s evident that it can be a task to clean up the data, standardize them, and make it suitable to support predictive analytics use cases.
Inaccurate data
The accuracy of predictive analytics boils down to data quality and not quantity. It is possible to tame large data sets and make them usable. But, inaccurate data is more problematic and, unless detected, could create wrong projections and lead to serious process errors. Imagine the loss a company could incur, going after a misleading demand prediction report and stocking up wrong supplies.
This shows the importance of accurate data and of how the lack of it could lead to faulty models.
It mainly happens with logistics and supply chain units with poor data management and collection practices. You could start with a brief data assessment and find out how you could fix issues with master data management.
Identifying the right expertise
Building predictive analytics models requires a multidisciplinary approach - a combination of data engineering, science, and AI skills along with a sound domain knowledge is a necessity. Other than business understanding and alignment, it also takes designing compliant and scalable solutions. There are also other considerations and support requirements you might need from your provider like the following.
Being aware of ethical requirements and liabilities to design responsible AI solutions.
Should be able to handle the data preprocessing part and preparation part to derive maximum results.
Hands on experience to derive the right solution and approach for your problem along with selecting cost-effective tools and infrastructure.
Periodic communication and support from the provider.
Post deployment support and model tuning for maintaining the accuracy.
Smoothly work alongside your current team to fast track development and deployment processes.
If you want to build customized predictive analytics for logistics problems, use the above as a checklist to find the right talent team to build solutions.
Data privacy
Data privacy is a pressing issue for logistics companies. Since most predictive analytics use cases in logistics involve sensitive information like customer data, sales details, etc, it’s important to see if the solutions meet legal requirements. Any breach or cyber attack could put the data into risk. The onus is on the developer to account for ethical, privacy, and bias factors while designing a robust AI solution throughout every lifecycle.
Also, these privacy policies and industrial regulations are never constant. It requires regularly keeping up with the changes and updates in the legal landscape and making necessary updates on your application to gain your customer and vendor trust.
How using predictive analytics may improve logistics operations?
eCommerce is at its all-time peak, because of which logistics, warehousing, and freight companies must take up the extra load. This is where modern predictive analytics can help ease the load.
From Walmart to Amazon to FedEx, every company relies on predictive analytics to optimize their inventory and supply chain management. They have turned their freight management well-planned and smooth-running by relying on technology like AI and IoT and data. This has also positively impacted their bottom-line, enabling them to deliver amazing customer experience despite the changing workloads and seasonal factors.
Supply chain predictive analytics have brought the following changes successfully.
Can manage demand fluctuations with ease
Less inventory holding and wastage
Efficient vehicle routing that leads to low fuel costs and fast deliveries.
High customer satisfaction as their demands are met on time.
Leads to smart inventory management - right products available at the right time.
Increased lifespan of equipment, vehicles, and other resources.
Has utmost control over procurement of supplies and vendor relationships
Final thoughts
Predictive analytics sounds highly promising for the logistics and the supply chain sector. But, you can’t build that in one day or a month. It takes years of carefully managed data and expert AI and machine learning teams who can build predictive models. If you are looking for one such team with a good grasp over the logistics landscape, sign up for a free discovery call with us. Talk to our data science and AI consultant and decide if your logistics company is ready to adapt predictive analytics. We could run a detailed assessment for you, finding the right data solution that aligns with your current business needs or future goals.