How to build your AI strategy?
A brief guide for CIOs and CTOs on building AI strategy for businesses from scratch. Everything you need to implement valuable AI technologies into your business at optimal costs, timeframes, and resources.

Thulasi
July 26, 2024 |
7 mins
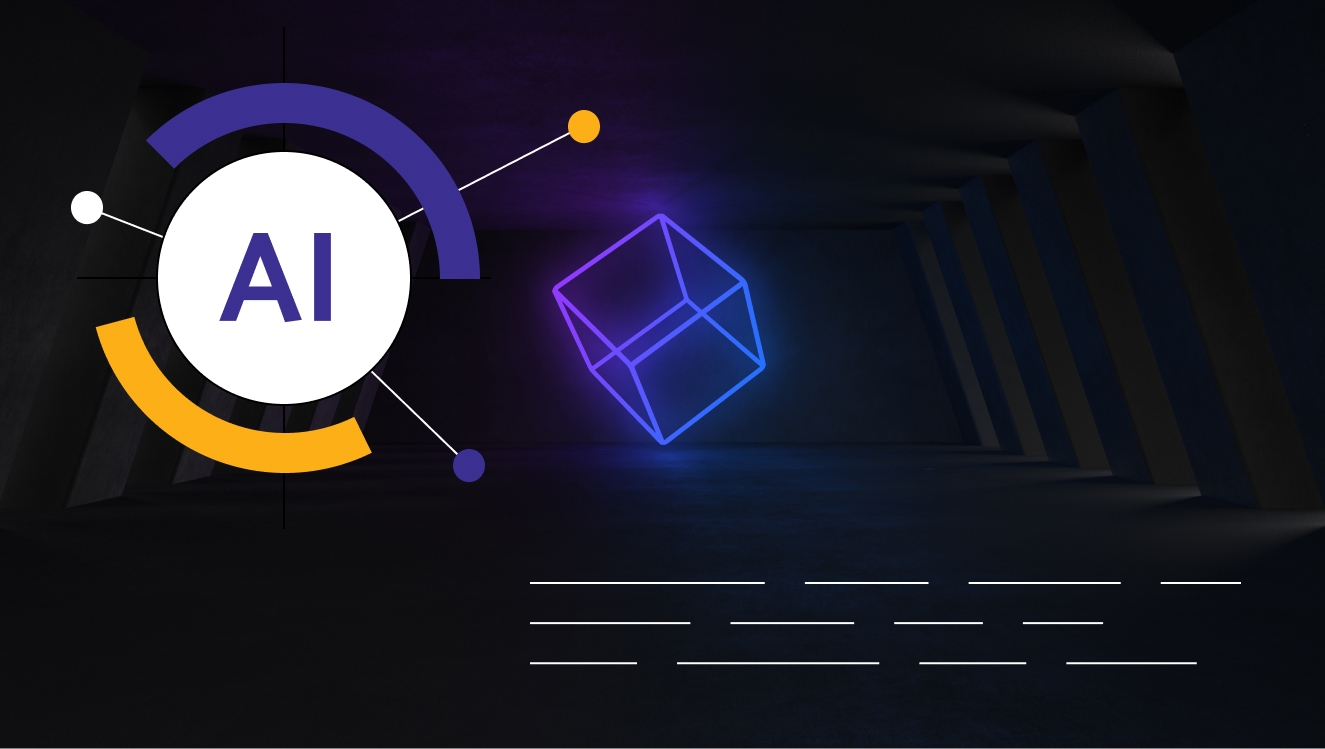
Why is an AI strategy important?
Businesses invest in AI for all reasons - to improve customer service, to automate internal processes, to strengthen cybersecurity, and many similar reasons. Close to 77% of companies globally are already using AI or thinking about incorporating AI in some forms.
The starting point to a successful AI implementation is a thorough AI strategy.
A strategy can ensure that your initiatives align with business goals and reap maximum value out of your investments, with given optimal utilization of resources. It can be a guiding point, providing a clear roadmap with KPIs and milestones to measure how effective you are all the way along.
What should a good AI strategy do?
A good AI strategy must cover the following.
Clear and detailed objectives of what you want to achieve from the AI initiative.
data infrastructure examination to find the quality of your data and how it can support AI products you build.
Identifying organizational readiness and finding areas for training and improvement.
Ethical and governance guidelines for building AI products that are responsible, fair, and meet regulatory standards.
Tools, technologies, and frameworks required for AI/ML development
Procedure for continuous monitoring post deployment to ensure that you achieve maximum outcome and ROI.
Instructions on stakeholder management: the roles each team and stakeholder will partake and the modes of communication.
The simplest way to assess and approach an AI strategy is by evaluating the four pillars of the AI strategy you built. The four pillars of AI strategy are data, tools and technologies, people and skills, and governance. Tending to these four categories and ensuring balance among them is your key to a well-thought out, success-driving AI strategy.
Build your AI strategy - step-by-step instructions
Finding success with AI
According to Gartner, nearly 85% of AI projects fail. Reasons why these AI projects fail are the inability to integrate AI into existing systems, data quality issues, internal politics, lack of skill, and security and compliance challenges.
And, VentureBeat suggests 87% don’t make it to live, which tells the widening gap between AI development and thriving deployment.
But there are success rates of AI implementation too—22% of companies that incorporated AI report at least 5% of earnings (before interest and taxes), that’s attributable to AI. Also industries like finance, healthcare, and technology find high rates of success with AI compared to other industries.
While an AI strategy cannot be a foolproof way to find success with AI, it can still show you the right path, leading to measurable results.
Also note that one size does not fit all. That’s why we have attempted to keep the steps as generic as possible. Using this, you could create and curate a rough roadmap suitable for your business.
Step #1: Set clear objectives
Set SMART objectives - that’s the quick way to have clear, actionable goals once you decide to start an AI project.
S - Specific. Helps you to be clear about expectations from AI systems.
M - Measurable. The outcomes should be measurable. Example. 30% cost reduction.
A - Achievable. This makes sure that your goals are attainable with the resources and timeline you have.
R - Relevant and realistic. AI goals aligned with current business problems you face. Example. Reducing customer churn, improving service availability, etc.
T - Time-bound. Set reasonable timelines within which your company should achieve the goal.
The above step identifies the problem statement and the time you have to sort this out.
Finding this SMART goal requires conducting regular meetings with stakeholders to identify key pain points to fix using AI.
Step #2: Develop AI strategy roadmap
You require an AI strategy that matches with your above business requirements. To prepare that, you need to start with a roadmap, the key component of any strategy. Your AI strategy roadmap is your reference point throughout the AI project, guiding you with the necessary information on your current state and the goal you want to achieve. It talks about everything - testing your current data availability, quality to compliance check to finding the right talent.
Step 2.1 - Feasibility analysis and data strategy
One of the reasons to ensure successful AI project implementation involves conducting feasibility analysis. Once you have the goal and an AI use case that aligns with it, you should perform feasibility analysis. This includes assessing:
data availability, technical requirements and feasibility.
Operational logistics
Possibility of risks and ethical violations.
Audience opinion and market demand for the product
Current tech stack and any gap that needs to be addressed.
Tools and technologies you will require that can support you scale your AI project further.
Team selection for AI development - finding people with the right skills to work on the project. (explained in detail in step 3).
Based on the available technical resources and feasibility, select one use case or idea that your company can prioritize now. If you are just starting with AI, it’s better to focus on high-impact, low-cost models for high success percentage with AI implementation strategy and build momentum thereon.
Step 2.2 Ethical guidelines
AI strategy must dive into ethical guidelines for ensuring responsible and fair AI usage. This can be new for many CIOs especially if you are new to AI initiatives. But, developing comprehensive ethical guidelines for AI is as simple when the following boxes are checked.
Bias and fairness: Many AI systems are accused of showing discrimination and bias towards selective people, picking unexplainable traits from training data. Ethical guidelines should talk about bias detection mechanisms and audits to ensure your AI systems treat everyone equally.
Accountability: What if the system performs errors? Ways to audit and resolve them and who will resolve them.
Security, confidentiality and data protection: Are you getting consent from the respective individuals or stakeholders for using their data? How strong are the security measures against data breaches and attacks and frequency at which data audits will take place.
Compliance: Are you following every recent law regulation related to data and AI?
Not overstepping on organizational values: How inclined are the AI use cases to company’s value statements? Can the system have any negative consequence over employees, clients, management, or public?
Step #3: Find the right vendors
You have the problem statement, project requirements, and feasibilities in hand. It’s time to find a vendor who can support developing the model for you. If you have an inbuilt data science team, you might still need vendor support for additional resources, tools, and technologies.
In either case, here’s what you have to look for in an AI and data science service provider.
Proven track record and successful implementation of similar projects
Case studies and additional supporting materials.
Data scientists’ availability and proficiency in AI, data analytics, and ML algorithms.
Working style, culture fit, and compatibility with the vendor team members.
Extended support and ongoing maintenance of the project.
Data security and regulatory compliance standards of the vendor.
Client assessment using a small trial project or a POC by the vendor.
Your strategy should account for all the above factors and how your company will give a fair chance and consideration before selecting a vendor.
Step #4: Monitor and iterate
AI strategy doesn't end with project development and deployment. It also covers what happens beyond and how your organization will adjust to the new system.
Create an AI strategy that explains what you should do post delivery of the AI project. Make sure that your strategy covers the following areas.
Evaluating performance KPIs to understand the ROI and success rate of the project.
Gaining real-time feedback from end-users and sharing that with the development team.
Identifying potential loopholes and areas of improvement and communicating the same to the team.
The time period the team must take to work on the fixes and improvements.
Impact and bias assessments and how frequently they will happen.
Performing iterative improvements to the product and measuring its effectiveness.
Above all this, AI projects require continuous monitoring and retraining to sustain their accuracy levels. So, your strategy must have an answer to what kind of data collection, preprocessing steps your team should follow for this.
Benefits of having an AI strategy
With increasing failure rates and ballooning development costs, AI strategy becomes incumbent for CIOs. Some other reasons why you need an AI strategy for business.
Make better decisions: If we go back to why AI projects fail, one point you will notice is how the cumulation of poor decisions ruins the project beyond repair. Be it deciding the objective or finding where to spend. A company that expects clear and effective decision-making would always start with a strategy.
Detailed insights: There is a difference between a problem statement and an actionable plan, providing detailed insights on objectives, data, governance, and everything. It makes it easy for both internal teams, vendors, and other stakeholders to work alongside each other, chasing one goal.
Resource optimization: With everything written down, the path becomes clear and so as the concurrent steps, needed resources and investments. You could wrap up your AI project much faster with fewer resources and investment than estimated.
Competitive advantage: Being able to achieve more success with AI gives you the competitive advantage, especially when everyone around is working on similar use cases. Such cut-throat competitions require you to be agile and precise with your development activities, and a strategy seals the outcome.
Less confusion. More work done: An AI strategy clearly communicates to every stakeholder of their roles and involvement. Giving the much needed clarity, this avoids unnecessary chaos, leading to more productive and hyper fast development.
Am I ready for AI adoption?
Your competitor is building an AI component. Doesn’t mean you should do it too. It’s too risky to start with an AI strategy or development without checking if your organization is ready for it, given that the project can cost in millions.
Nevertheless, you could easily determine your AI adoption stage by assessing these five pillars - strong need for AI, data, infrastructure, employee talent, budget, and compliance constraints.
Check out the AI readiness assessment questionnaire prepared by our senior data scientist. It only takes a minute to fill out the simple questions and find your assessment score.
If your answer is ‘yes’ to the majority of the questions, your organization shows a high level of readiness for AI projects. If you are left with ‘No’ for more questions, then it’s good to approach an experienced data scientist for a deep assessment. This can unlock many opportunities for you that solve your problems, keeping the restraints in consideration.
At the same time, you should know how these restraints affect your chances and what can be done to overcome them. For example, some companies face challenges with culture fitment where employees find it harder to adopt. In such cases, training the employees and preparing them for upgrades should be prioritized more.
Final thoughts
‘How to implement AI & ML in business’ and ‘how to build ML models for that’ are some of the burning questions for many CIOs even today. As a data science and AI solutions provider, we have explained the starting steps you must take for successful AI implementation. As you evaluate factors like data availability, infrastructure, talent, clear objectives, resources, and ethical considerations, you can be fully prepared before you start. If you require more wisdom into the process, you could reach out to us for AI consulting and development. We will work with you to find your goals and share feasible solutions. Check out our successful AI and data science projects here.