Data quality best practices
Data quality decides how good your decision-making is - Gartner says. Get answers to what is data quality, how to ensure data quality, why it is important, and some data quality best strategies you could implement. And everything else you need to know about data quality.

Suresh
September 5, 2024 |
8 mins
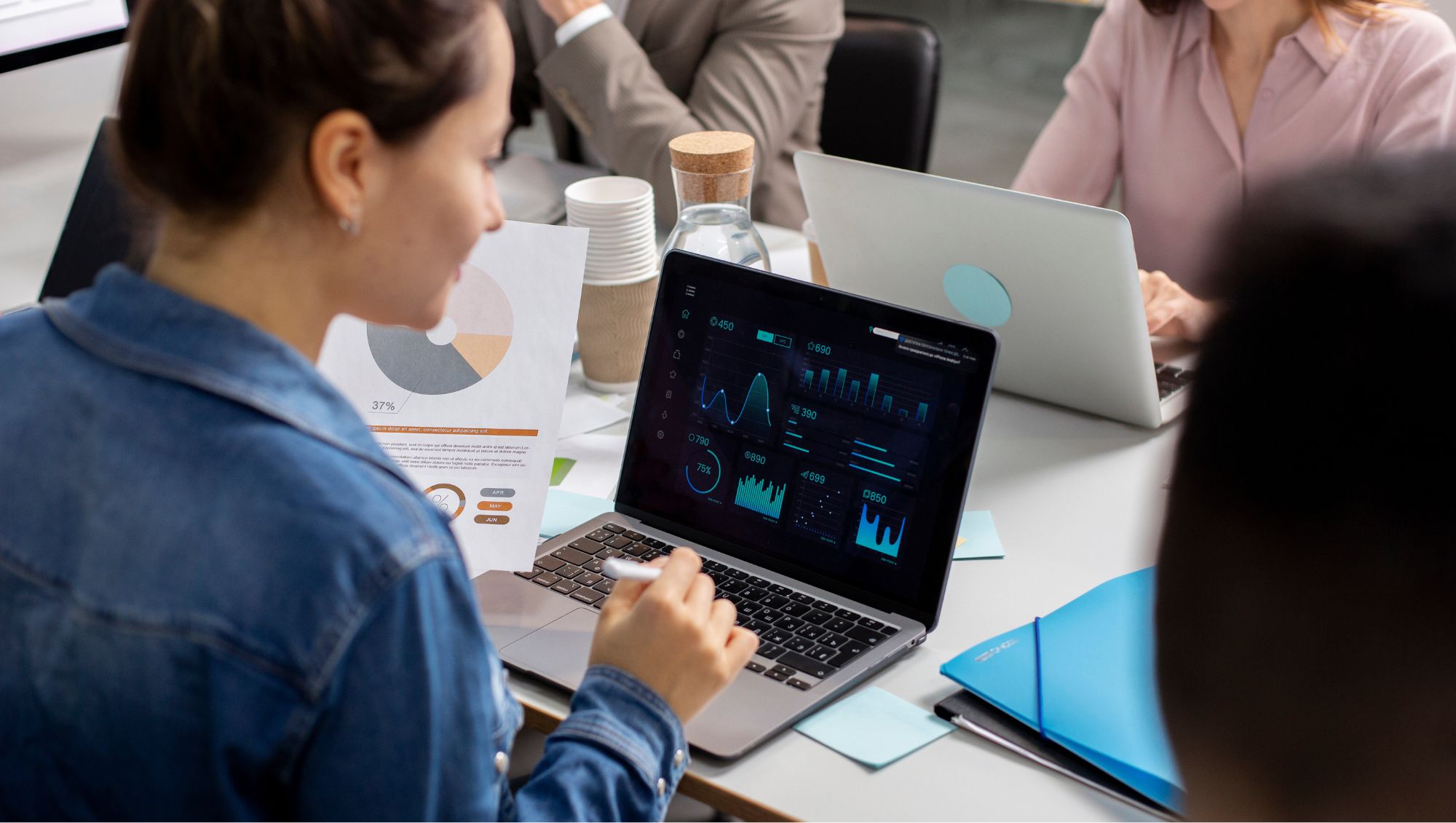
What is data quality and why is it important?
Data quality is the state of data at which it is accurate, complete, consistent, and reliable. There are many other parameters too that define good data quality—timely availability, understandability, ease-of-access, whether it's fit for purpose, etc.
Data quality is an important part of data management and a serious problem many data teams work hard to solve.
Now the question is why it matters. Data quality matters, as it could directly impact the effectiveness of business operations, decision-making, and strategic measures. More than 25% of a survey’s respondents have noted that data quality issues affected 75% revenue. Shows the impact of data quality on the bottom line of a business.
Other reasons why data quality is important
1. Risk management: poor data quality is a risk on its own. Besides, it could not let you foresee business risks on the way. On the other hand, high data quality generates accurate insights, gearing you up for any credit risks, economic turbulence, or regulatory reporting challenges.
2. Good decisions based on facts: Organizations with good quality data gain trust in their data and make confident decisions, putting less effort into strategizing, planning, and brainstorming.
3. Reduced costs: no budget required to find and troubleshoot data quality issues, nor will you make poor decisions due to incorrect data.
4. Acts as a growth catalyst: Building a new product, expansion, or other growth initiatives become successful with accurate data insights.
5. Seamless data integration: With multiplying data sources and enterprise applications, data quality is needed for instant integration.
6. Better analytics and BI: High quality data becomes much more actionable and easy to consume by the end users.
Data quality dimensions
Data quality dimensions are attributes or characteristics you can use to estimate data quality. Imagine this as a crystal stone with different angles that reflect how good or bad your data is. Those dimensions that decide your data quality are accuracy, completeness, consistency, timeliness, validity, and uniqueness.
Accuracy
This side represents how accurate the data is and how it represents something like a real-life element coming from a verifiable source. Think of a verified customer phone number or email address. In this case, your data quality is good and your marketing/sales team could reach customers easily.
Completeness
This dimension is whether your data includes every necessary information or not. You request customers for a product feedback and it comes back with a missing field - probably name or contact information. Then, it can be considered incomplete. But, completeness can change for different entities. In the same form, an address or landmark might not be a necessary information, but the rest of it helps and can be assumed as complete.
Consistency
This dimension denotes the sameness of data across many sources. Consistency issues can be of many types - from basic formatting errors in spelling issues to entirely unmatched records of two or many systems. For example, the same customer can be ‘Ken’ in one record and ‘Kennedy’ in another. Or, one system might reflect an old address while the other carries updated data. In either case, this is an issue and reflects poor data quality, affecting data usability.
Timelines
Timeliness is the dimension that talks about the availability of the data when you need it. Real-time or near-real time, do your business users get access to reports on time? Then, it is timely. And, if it’s not, then this category will be invalid in your data quality dimensions.
Validity
Every data must follow a specific format to be qualified as valid. That’s what this dimension of data quality denotes - whether your data sticks to the assigned format everywhere. One example is writing dates. Are all dates written in the same format chosen, date first, month, and then year? (or, as per the common format followed in your region). Or, does the zip code contain the right number of characters? If yes, then the data is valid, improving your data quality score.
Uniqueness
Uniqueness as a dimension represents the absence of duplicates in your dataset. A record containing two different versions of the same user data is duplicate data. This scenario is usually common in customer records. Uniqueness is about maintaining a single version of data, serving unique information.
Data quality best practices
Data quality is a continuous problem. The only way to tackle this and improve data quality is following the best practices for data quality like data cleaning, continuous tracking, creating awareness, and setting the right quality standards.
1. Ensure data governance
Analyzing current data governance is where you should start. Data governance helps you with policies and procedures for maintaining data quality across the company.
If you have a data governance board already, collaborate with them. Review how strong current policies are and how the organizational data adheres to the standards. If you don’t have governance policies, it’s time you create one. There are many easy-to-use governance tools like Collibra, Precisely, Microsoft Purview, etc. Select your tool, create your governance framework, and decide how data management must happen in your whole company.
2. Data cleansing
Clean data means quality data, and this is directly related to many data quality dimensions, like accuracy, consistency, etc.
While performing data cleansing, remove duplicates, standardize formatting, and fix any out-of-date information. And, this isn’t a one time event. Keep data cleansing as a regular activity to improve data quality consistently.
3. Establish data standards
If your company doesn’t have data standards, start setting up one. Data standards mean setting up procedures for data collection, transformation, storage, and usage. It can be about standardizing formats, setting acceptable range of values, and other validation rules.
These sets of standards can not only help with managing data, but also with the periodic cleansing process you set up.
4. Continuous tracking and improvement
Data quality management is a continuous process, not a one-time effort. You should establish a culture of continuous monitoring and improvement. You could create a team and assign members their responsibilities to address data quality issues. The team can be responsible for data quality measurement and auditing.
Other than fixing existing issues, forecasting and preventing them is much better, reducing cost wastage and ensuring business continuity.
5. Educate and train your team
Ideal data quality is not only the data team’s responsibility, but every business user and consumers’. To maintain data quality, you should train and engage stakeholders beyond data teams. Discuss and engage with different teams to make sure that your data quality initiatives are effective and align with their requirements. Conduct training sessions and assessments to evaluate whether users understand the healthy data quality practices and consumption.
6. Data profiling
Get started with data profiling to analyze and understand your data structure, content, and quality. It’s one way to find out any inaccuracies and anomalies to ensure that your data quality is up-to-date. Other than finding out data quality issues, it also does structural and content analysis for more efficient integration, cost savings, and better decision-making.
7. Create reports and dashboards
Visualization is the easiest way to track data quality trends over time—gaining insights on deteriorating or improving metrics. A data team can set up periodic, automated reports on data quality metrics like completeness, validity, accuracy, etc. and take timely actions upon problem zones. Visualization also motivates the involved teams and other stakeholders and helps with data quality audits. With modern data visualization technologies, you could even set up real-time data quality dashboards to track elements of data quality, department-wise, region-wise, and application-wise.
Data quality challenges
While ensuring data quality, you might encounter many data quality issues, too. Some common data quality issues and how to handle them.
Duplicate data
Most common data quality issue is duplicate data. Duplicate data happens when the same data is present many times within the same record or different records. Complex data architecture with many data integrations and merging often has this issue. If you don’t fix duplication issues, you will be left with unreliable insights, skewed results, and high storage costs.
Routine deduplication process along with data audits is the fix to resolve duplicate data issues.
Inconsistent data
Inconsistent data is a common problem among data teams, which occurs due to wrong and mismatched data formats. For example, one record might be following “MM/DD/YYYY" and “DD/MM/YYYY". This is a classic inconsistency case.
This data inconsistency mainly happens due to human errors, lack of validation rules, or integration across cross departments. You can fix this issue by establishing data standards across your organization.
Hidden data
Hidden data can be a problem to deal with, as these data sources are often siloed and unidentified sometimes. IBM says that 80% of supply chain data is hidden and the same story lies in every industry. With large amounts of data being hidden, it’s impossible to draw accurate conclusions from data.
Data silos and hidden data also lead to inconsistencies and duplication, wasting resources for everyone and hindering opportunities to cross-refer. You can fix the hidden data issue using a centralized data management strategy like data fabric or a centralized data warehouse for overall organizational data with domain-specific data marts.
Human errors
Human errors happen when there is manual intervention. It can be basic like a typo or a misentry, to huge things like an integration error or compliance risks. It might sound insignificant, but it can lead to poor analytics, incorrect processing and billing, or other expensive mistakes.
The only way to fix human errors is to employ data automation, keep validation checks, and insist on training for data teams.
Outdated data
Outdated data refers to the data that’s not refreshed on time. This data might not serve value and only hike your storage expenses. It can be highly misleading also, causing wrong inventory orders, customer targeting, or mis-priced products and services. To solve this problem, you can go for automated data updating or scheduled data feeds that perform an incremental update of datasets and updates without redundancy.
Final thoughts
Data quality can be daunting no matter how far along you are in your data journey. It can be a hindrance to your product development, customer engagement activities, other business operations, and AI initiatives. You will need more than data quality best practices when you are starting. A data quality strategy that’s curated for your organization is important. It can be a guiding roadmap for starters and show how to get the show running without worrying about data quality. Talk to our data strategist today to analyze your data quality maturity levels in less than an hour.