Data analytics maturity models
Assess how far along are you with the data analytics maturity journey. Learn about the stages of data analytics maturity, framework, components, and tools and techniques, along with how to get ahead on the analytics maturity curve.

Jagadeesan
Dec 3, 2024 |
8 mins
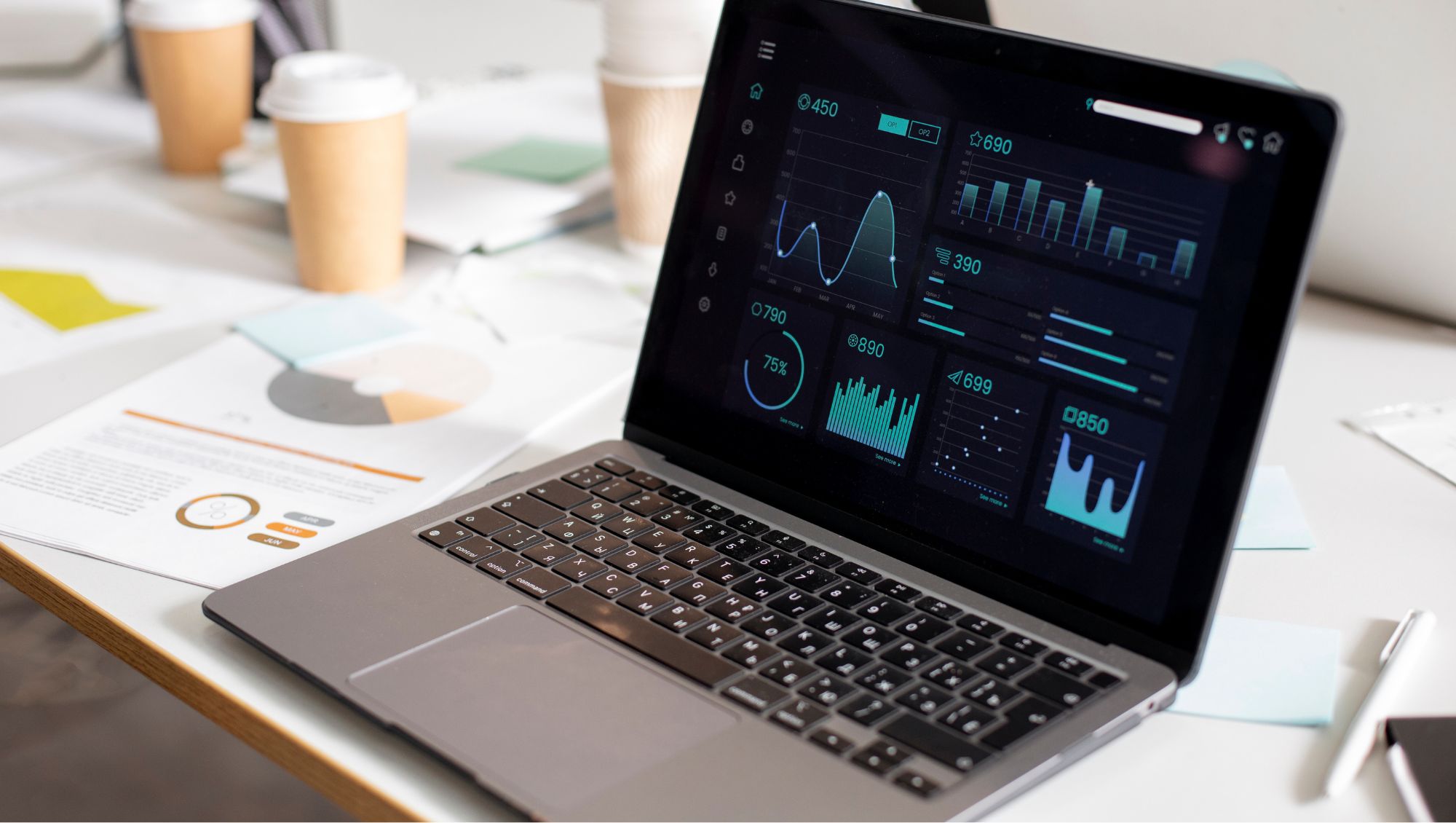
Data maturity model explained
Data maturity model is the framework you can use to measure your organization’s ability to derive value from data. It defines how good your company is with data management, robust analytics systems, AI and other use cases. A company with high data maturity is someone with streamlined data processes, analytics systems, and data-centric culture in the middle of it all, extracting maximum value from data.
Characteristics of data maturity
Some factors and questions that decide a company’s data maturity levels.
Data governance and quality: are there strong data governance policies in place to ensure that the data is accurate, reliable, secure, and timely?
Tech and tools: are there modern tools in place for data and business teams to process and interact with data - from cloud storage platforms to BI to self-service analytics.
Integration: how interoperable and flexible are the data and teams? Are there silos, separating functional areas, preventing holistic, multi-dimensional views?
Analytics and AI: Is the company using advanced analytics and predictive systems to stay ahead of trends? How well the company is leveraging AI and ML to automate workload, predict future trends, and rule out uncertainties?
Cultural alignment: how well is the data literacy rate across all levels of the organization? Is everyone adept enough to make data a part of their day-to-day work and decision-making?
Data analytics maturity model assessment decides where a company falls in the data analytics maturity curve based on their current tools, analytics workflows, and data culture.
Analytics maturity model stages
There are four levels in analytics maturity models - descriptive, diagnostic, prescriptive, and predictive. But, there are many other phases in between too, like the foundational stage, cognitive stage, etc.
Foundational stage
Data is scattered across systems and is unmanaged.
No or limited data tools are present and decisions are made based on intuitions and not based on data. Or, in some cases, analytics are carried out on an ad hoc basis with rudimentary level tools like Excel.
Lack of policies and procedures on how to handle or data.
Example: generating reports using spreadsheets upon request from business teams.
How to progress from the foundational stage to the descriptive level? - Implement basic reporting tools like Power BI, Qlik, or Tableau. Start organizing data after an in-depth cleansing and centralize its storage using data warehouses.
Descriptive
Descriptive is the second stage, aka developing stage of analytics maturity models.
Presence of basic data tools, dashboards, and reporting systems.
Organized and managed data, but not to a great extent. Fragmented and poorly integrated data sources are still there.
Every team’s analytics requirements will be met, but they all function in siloes.
Example: Data analysts create monthly sales or marketing performance dashboards, aggregating two or three data points. These reports might still lack detailed insights, but have sufficient information to know what’s going on.
How to progress from the descriptive stage to the diagnostic level? - Connect data from multiple sources and integrate them into one centralized location, preferably a data warehouse or a lakehouse. Review your data governance policies and make improvements.
Diagnostic
Diagnostic is the third stage of data maturity models, also known as the proactive stage.
Companies have systems in place to process and store historical data and derive insights from them. These insights are deep enough to show trends, patterns, and root causes behind lags and performance, if any.
There are standardized processes for managing data quality and governance.
Data is integrated for more accurate and reliable cross-functional analysis.
Tools used: ETL, data lakes, warehouses, and BI platforms, automation, collaboration tools, and SQL.
Example: A company uses sales performance dashboards to find out a minute dip in sales in a particular region, correlating with marketing spend and campaigns.
How to progress from the diagnostic stage to the predictive level? - start using cloud-based platforms for more real-time data access. Find out AI/ML use cases that can solve business problems and implement them.
Predictive
Predictive is the advanced stage in the data analytics maturity model. Companies in the predictive stage use analytics and predictive models. These models could be ML based or statistical, but they use historical data to predict future outcomes.
Advanced data processing tools and techniques are present to make near-real-time analytics possible.
Tools used: cloud storage and processing tools, visualization applications, predictive modeling or other AI-based models, and self-service analytics applications.
The sales team of a company uses an accurate demand forecasting model that predicts future sales based on current and past sales, economy, weather, and other external patterns.
How to progress from the predictive stage to the prescriptive level? - Make data and AI a part of your team’s everyday workflow, not a standalone application that generates insights. Pay more attention to data literacy and culture to improve user-data interactions. Aim for continuous improvement.
Prescriptive
Prescriptive is the highest level in data analytics maturity models. Predictive insights are available in real-time, which are embedded in workflows.
Companies with prescriptive analytics levels treat data as their strongest asset and have exceptional governance principles to ensure data quality and usability.
Analytics is a part of every work and workflow now, data is institutionalized as the basis for decision-making, and the benefits of analytics in business are seen by all.
Tools used for prescriptive analytics: IoT, AI/ML applications, advanced analytics, etc.
Example: Automated optimization of product pricing based on current demand, competitor strategies, and many other conditions that play a role in sales.
Importance of data maturity models
Data analytics maturity models are your way to find out existing analytics processes and tools and how to improve them. It can help you tap into your strengths and make it better or work on your weaknesses.
It’s hard to achieve great progress overnight. But step-level changes are doable. Data maturity model assessments show you where you stand and where you can go next.
Data isn’t a separate unit, but a part of your business assets. Using data maturity model assessment, you could align your next data initiatives with business goals, making improvements in both. For example, finding the need for predictive capabilities to prevent lost sales opportunities.
Data governance and security are crucial aspects to take care of, as your business data scales further. DAM assessments directly help you improve and standardize these policies to meet regulatory requirements.
Final thoughts
Data analytics maturity model assessment is your roadmap to become fully data driven, extracting the best value from data. By adopting and implementing a data maturity model with the help from data analytics consulting companies, your data awareness levels increase, encouraging experimentations and facilitating innovations in all areas of the business. The model brings a structure and organization to your plans, making it easier to drive digital transformation initiatives to success.
Start with a discovery call with our data experts who could work with you on this assessment, identify your current stage, and share realistic recommendations based on your business goals.