AI use cases in manufacturing
How AI plays a role in the manufacturing industry and what are the real-life use cases? How can manufacturers use AI to tackle major issues like productivity, quality control, regulatory compliance, maintenance, etc? Dive in to know how manufacturing companies are using AI and growing faster than ever.

Thulasi
April 6, 2024 |
6 mins
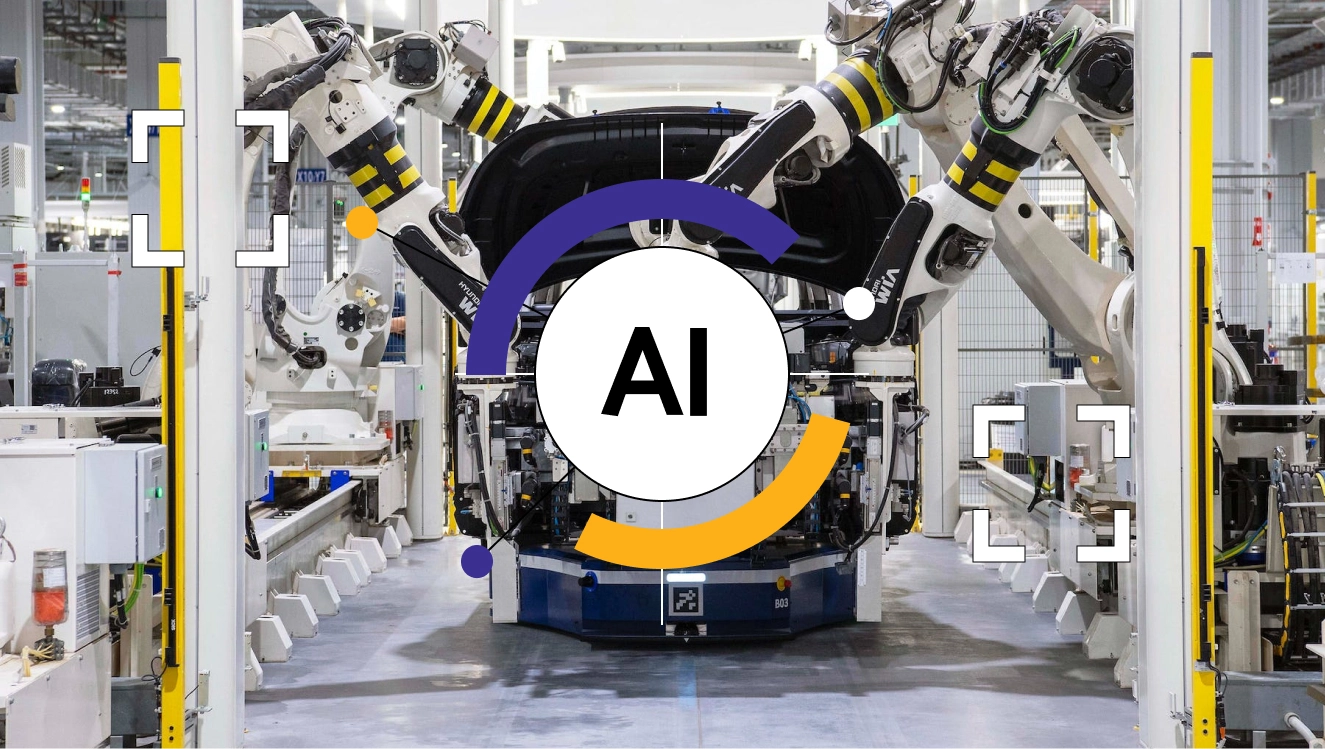
Top 8 use cases of AI in manufacturing
A study from Microsoft highlights that manufacturing companies in the US that use AI perform 12% better than organizations that don’t use it. This 12% improvement proves the impact of AI in the manufacturing industry on solving complex problems that directly boost a company’s performance and growth.
AI is used in manufacturing in the following ways:
Quality control: Using computer vision to detect defects and fix them and bring consistent product improvements.
Workflow optimization: Make automated schedules and let optimal resource utilization happen.
Supply chain management: Improve delivery times, reduce disruptions, and get started with real-time tracking.
Energy efficiency: Optimizes energy consumption and lower down fuel costs.
Worker safety: AI-powered maintenance to identify hazards ahead and prevent accidents.
Some artificial intelligence in manufacturing examples includes predictive maintenance, assembly line optimization, cobots, and real-time quality assurance systems.
With multiple historical data and records, manufacturing companies can surely incorporate these artificial intelligence solutions for manufacturing.
Reducing downtime through predictive maintenance
This is about using AI to predict when a machine will likely fail and fix that in advance to minimize downtime, prevent safety hazards, and reduce operational costs. Manufacturing units typically rely on reactive or scheduled maintenance. Reactive - fix when a machine gets broken. Scheduled - fix frequent sessions for maintenance activities.
But there are other exceptional cases that can also deteriorate a machine’s health and operability—increased workload, fault-prone spare pieces, objects getting stuck in the machinery, etc. This is where predictive maintenance helps.
It constantly analyzes the condition of the machinery from data collected through various sensors and IoT. These sensors are to perform wear-and-tear analysis, oil analysis, acoustic monitoring, vibration or heat measurement, and more. Any anomalies detected in the values, it raises alerts leading to proactive fixes before a fault or hazard occurs. For example, PdM can detect excessive vibration on the machinery which gets communicated to the floor support staff, allowing them to identify the root cause and fix the glitch within minutes.
By tracking parameters like temperature, speed of the machine, vibration, and other custom values, predictive maintenance can track its depreciation rate.
PdM bridges the digital and virtual world enabling factory supervisors to have a reliable maintenance workflow while increasing the remaining useful life of equipment.
Some machine learning algorithms for predictive maintenance in manufacturing includes regression models, reinforcement Learning, neural networks in deep learning, anomaly detection, and SVM.
Detect defects in quality assurance accurately with AI
Quality is a key tenet for manufacturing companies for various reasons - to reduce wastage, to ensure consumer satisfaction, standards adherence, and many more. This is why they have stringent and complex quality control procedures. Yet, performing this manually has many challenges like challenges in tracking defects.
AI-based quality assurance systems can accomplish this with more accuracy and ensure that only products that fully meet standards are sent out to customers.
Here is how it works. Images and videos of products captured from all angles are fed to a combination of deep learning and computer vision algorithms. It’s trained on both defective and non-defective pieces. The algorithm later understands how the product should be and compares it with every manufactured product with a high scale of precision.
If any defect is noticed, the whole manufacturing process stops until the root cause is found and fixed, separating the defective product. Summing up, Al & ML use cases in manufacturing help you prevent defects in advance, identifying potential issues with machinery that may cause defects in products.
Many leading companies like BMW, Canon, Boeing, etc already use AI as a part of their product manufacturing lifecycle to ensure quality control.
Learn more: AI in healthcare
AI to tracking fleet vehicles & do route planning in logistics
From accurate demand predictions to warehouse management to route optimization, the supply chain area can be planned and managed better with AI.
Moreover, AI can be used for warehouse spacing and shipping optimization so you can store and transfer more products at reduced costs.
This also applies to the transportation process to find out better routes and product combinations to transport to the relevant warehouse or storage facility. This takes the stress out of logistics planning, helping you transport the right level of required goods to where it’s needed at low fuel and labor costs.
Fleet management is another biggest challenge in the supply chain, requiring intensive manual planning and coordination with vehicle drivers/owners. Oftentimes, companies spend a lot on transportation with very little information available on what went wrong. AI-driven fleet management can help power fleet managers with real-time analytics and insights on transportation. It can share the fastest and most efficient route analyzing data like weather, traffic, load, vehicle condition, and more. This integrated operation ensures fast and secure transportation, improving productivity and reducing costs.
While on the topic, self-driving autonomous vehicles are also on the rise. They can deliver goods on time, share real-time updates through connected sensors, and reduce the possibility of goods damage or other unsafe incidents.
Altogether, AI use cases in manufacturing and supply chains bring in end-to-end visibility and ensure sustainability.
Learn more: AI in finance
Best way to manage stock inventory – AI inventory management
One crucial challenge for manufacturers is keeping their manufacturing in line with stock requirements and market demands. This helps them to not run out of stock or store too much than necessary.
With more and more factors affecting stock demands, relying on traditional forecasting methods may yield less accurate results.
Machine learning-based forecasting is the way to go here as it can consider numerous dynamic factors and share precise forecasting reports from time to time.
With the help of historical data on customers’ purchases and preferences, trends, seasonality, etc, manufacturers can predict future demands to match their production and storage. You can cross-refer data from different parts of the business—store shelves, inventory, and other storage areas, allowing you to understand the gap between demand and supply.
Another interesting AI use case in manufacturing is smart robots. Many inventory and warehouse units have already utilized such robots to move stock within warehouses, organize, lift, measure, and do other heavy labor.
This way, work gets done faster with less headcount and improved accuracy.
Reduce repetitive tasks with industrial automation
Industrial unit automation is an AI in manufacturing example, which automates hectic, complex, labor-intensive jobs using AI. This includes all the AI manufacturing use cases we have discussed above and more than that.
AI-based solutions can be helpful in many parts of an industry, helping with purposes like decision-making, energy utilization, and optimization, process optimization, etc.
Some examples include:
For instance, applying predictive maintenance to identify potential issues with machinery parts rather than processing it manually through scheduled maintenance.
Or using automated systems to detect defective pieces and separate them from the perfect products, further enhancing the quality assurance process.
It can also be about utilizing automated AI-based systems like cobots—which can perform labor-intensive and mundane tasks like lifting heavy weights, packaging, assembling, etc. These are collaborative robots that can safely assist humans in complex operations, particularly meant for risky work areas where employing humans is not possible.
One example of this is Adidas which has set up its automated, high-speed working unit called Speedfactory to scale up its manufacturing.
Cost forecasting for raw materials
The purchase price variance is a pressing budgeting challenge for manufacturers. It is the difference in the price they planned during budgeting vs what they paid while procuring the raw materials.
This is harder for manufacturers with multiple products and umpteen number of vendors.
An AI application can easily make this a manageable process and offer centralized insights on buying, raw materials pricing, and vendor management.
It can analyze your history of purchases, show a standard pricing model, compare prices across multiple suppliers, and group every required raw material for manufacturing a product together.
Generative AI – Can AI replace product design?
Imagine a design software that incorporates AI to create a next-level product design. Here is how it works. A designer will send their design instructions along with raw materials, specifications, cost, size, color, and any other parameters they consider.
The generative design software will come up with multiple design options that the designer can further enhance, test, or experiment with. Generative design shows how GenAI is used in manufacturing, generating numerous designs based on given constraints while learning from user feedback.
If manually done, this process can take up to months. The best part is that they can modify different parameters here and see how that affects the design prototype. This lets engineers move the best design forward based on what fits their expectations.
That being said, AI cannot entirely replace product designers but helps with automating tasks and creating large numbers of designs in a short time.
Generative design, being a prime application of AI in manufacturing, is already in use in many industries, like automotive, consumer goods, aerospace, construction, etc.
General Motors has ventured into this space to improve its vehicle design to be more eco-friendly and efficient. They went through an iterative design process, collecting inputs from multiple designers and stakeholders and transforming them into a 3d design prototype.
How to reduce power cost in industry? - dark factories is the solution
Dark factories, the most amusing AI use case in the manufacturing industry, can save tons of power required to light up factory spaces. As the name says, dark factories stand for no-light factory areas which are fully handled by robots and cobots. They wouldn’t require lighting to work, allowing the unit to operate in an entirely dark environment.
Though the topic is still debated and not fully experimented on yet, it shows a bright opportunity for companies to save up on electricity charges.
But it doesn’t stop manufacturers from experimenting with near-lights-out scenarios and fully automated factories.
Automated factories and robotic-powered factories are increasing in numbers, reducing the presence of humans on the shop floor. This way, AI can help manufacturers increase profits by taking complete control of production at minimized cost and wastage.
Take the example of Philips for autonomous factories, which has set up an electric razor manufacturing unit based on autonomous Case-handling Robot (ACR) systems with very few human workers only to manage quality assurance.
Learn more: How artificial intelligence helps in decision making?
AI factories
AI factories is a common term for advanced smart factories that utilize AI for automation, decision-making, and optimized production lines. They are well-connected by sensors and IoT devices, bring in real-time monitoring and alerting, and facilitate continuous learning and improvement. Benefits of AI factories are unparalleled quality, cost reduction, improved production, and less waste.
Example of an AI factory can be BMW’s smart factory which utilizes AI for predictive maintenance, quality assurance, and flexible production lines.
Conclusion
AI use cases aren’t new to the manufacturing industry. Capgemini’s research points out that AI has already been used by 51% of surveyed manufacturing companies followed by Japan with 30% and the US with 28%. It’s already dominating in many parts of the manufacturing sector from planning and operations to quality control to smart supply chain management.
Companies like Johnson and Johnson have adopted the digital twin technology to create the product manufacturing life cycle digitally in order to optimize the production environment.
It’s apparent that the manufacturing industry is familiar with both traditional AI use cases like inventory management, defect detection, forecasting, etc., and genAI use cases like generative design and chatbots.
So, this is the right time for you to invest in AI manufacturing use cases and revolutionize end-to-end manufacturing operations.
With datakulture by your side, you can start with the right partner who can help you with setting a strong data foundation till implementing the right data science and AI-based solutions. Take a look at AI in manufacturing case study, which shows how we helped them achieve lean manufacturing with reduced waste and low operational costs.