AI use cases for logistics industry
How does AI travel across the logistics sector and what are some interesting use cases? How are logistics businesses using AI to manage supply chain issues like fuel costs, labor management, high order intensity ratios, and more? Read the full article to learn more about logistics use cases that bring about supply chain disruptions.

Thulasi
Mar 15, 2024 |
6 mins
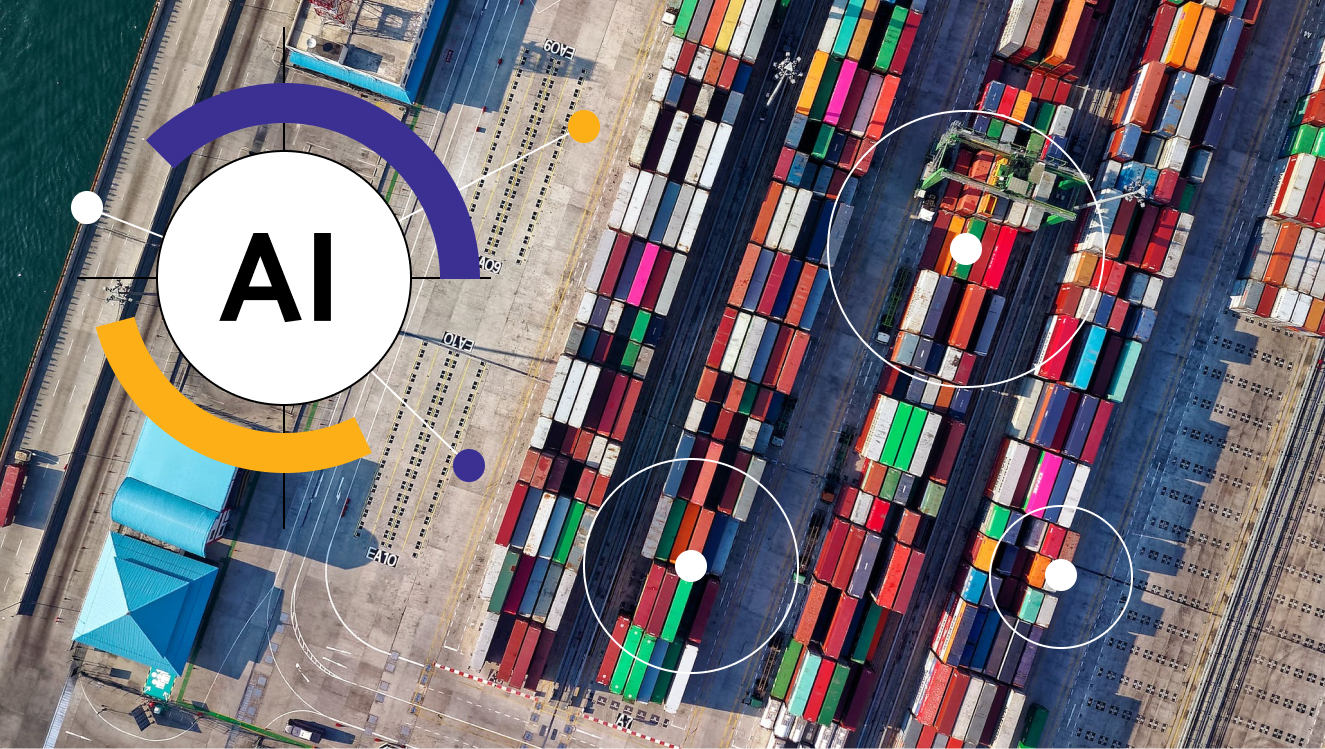
AI use cases of logistics
AI in logistics and supply chain opens the door to many interesting and smart possibilities, with use cases as unique as self-driving vehicles, fleet optimization, delivery drones, etc.
Benefits of AI in logistics include more streamlined and pick and drop operations that’s aligned with future demands, reduced spending on fuel, warehousing, and storage, and automated monitoring and tracking.
Some very good examples for artificial intelligence in logistics include demand forecasting, quality control of outgoing goods, autonomous vehicles, automated surveillance systems, and warehouse slotting recommender.
1. Demand forecasting
User demands change every day, making it challenging for logistics operators to perform last-minute transportation. Accurate demand forecasting allows them to know what product is needed, how much, and where, all ahead of time.
Typically, logistics and warehouse managers perform demand forecasting traditionally based on historical orders. But, AI can optimize logistics and how they meet demands through advanced forecasting that can handle huge amount of data and generate accurate forecasts. It can handle a huge amount of data and consider any kind of external factor and fluctuations. Their performance improves over time due to their adaptiveness and scalability.
With these forecasting reports, logistics companies can uncover more hidden patterns and streamline their supply planning. This helps them closely watch seasonal fluctuations, reduce transportation costs on unplanned transportation, and proactively keep up with changing demands.
Not only in transportation, demand forecasting also helps with production planning, storage management, and workforce allocation—all of which are pressing concerns in the logistics sector.
📖 Recommended read: AI use cases in Retail
2. Predictive maintenance
Forklifts, conveyors, elevators, material handling equipment—so much equipment in a logistics company that requires constant maintenance and care. Most companies either have scheduled maintenance or perform reactive maintenance, fixing once the machine gets broken. Both of these may reduce the lifetime of a machine or cause unexpected downtime, shutting down operations.
How to be proactive in taking care of this machinery and extending its remaining useful lifetime? A major example of AI in logistics, predictive maintenance, is the answer. This uses a combination of sensors, machine learning-based algorithms, and an alarm or a reporting system.
The sensors attached to the machinery capture information depending on the use case—heat, rotatory movement, acoustics and vibration, debris settled, etc. The ML algorithms are fed with a normal range of this data which also denotes the healthy operable conditions of the machine. If the algorithms pick up any spike in these numbers, it can intimate the floor personnel to look into it, suggesting relevant measures like giving the machine a rest or checking an anomaly object.
3. Route optimization
AI use cases in transportation are route planning and optimization, aimed at finding the most efficient route, utilizing the max capacity of vehicles—all while understanding in-depth traffic and routes.
Imagine you have 10 vehicles, 10 places to deliver each with 10 stops in between. The probabilities and possibilities can shoot up for finding the best route to deliver maximum products consuming the least amounts of fuel. AI-based route optimization can reduce computational complexities here so you can have better resource utilization and on-time delivery at reduced costs.
Manual route planning is usually made by humans considering inputs from drivers and past routes covered by them, which doesn’t take into factor dynamically changing conditions, ex. Traffic or roadblock. This can cause delays, hike expenses, and sometimes even lead to safety incidents. Even though it’s possible to club orders and deliver them together, it takes humungous human effort to carry through. So, traditional route planning doesn’t support route optimization like AI-based algorithms do.
However, machine learning-inspired algorithms can analyze historical delivery data, and GPS tracking data, plus real-time traffic conditions, weather conditions, possible roadblocks, and congestion to suggest a safe, fast, and reliable delivery route.
Since these ML models constantly learn and adapt to new data sets, the results will become more accurate over time.
📖 Recommended read: AI use cases in Finance
4. Warehouse optimization
From picking, slotting, and replenishing stocks to maintaining them at ideal levels to sending them on time, warehouse management requires AI-based and machine learning use cases in logistics.
Here are some of the ways AI helps here.
To cater to varying demands, inventories must ensure optimal safe stock levels. At the same time, the carrying cost for storing goods should be minimized. This can be computationally heavy to estimate for a large number of products and warehouse units, so AI-based algorithms can address this, keeping you updated on storage, demand, and reorder time until you can order the next batch.
Similarly, picking orders can also be optimized—routing or clubbing different picking processes together to reduce labor, time, and fuel costs.
Another paramount problem with warehouses is this—where to store them as slot sizes vary greatly. This problem requires more than product size and slot matching. By efficiently placing them, you can simplify picking and allocate less labor for that, while ensuring better storage utilization. AI-based algorithms can analyze demand, product characteristics, accessibility, and many other factors to find an ideal slot that guarantees the above benefits.
For combinatorial optimization problems like these, AI-based solutions work better given the higher number of factors to be considered and constraints to be met.
5. Quality control
Logistics often involve operations that might affect the external nature of goods—from cracks to breakage to colossal damages. Receiving such damaged products tarnishes the seller's reputation along with the logistics handler. Yes. Manual inspections take place before shipping. But, there can be misses and human errors, not to mention how time-consuming it is.
AI-based quality assurance systems along with computer vision to accurately detect defects and anomalies and automate quality inspection. To do this, the AI-based model is trained with a great number of pictures and videos defining product characteristics and specifications. Additionally, it’s fed with other product-related information like weight, size, colors, patterns, number of items, and more depending on the product type. The system learns how the product should be and grows its accuracy rate as it flags the defective ones before it reach the fulfillment center.
By combining quality control with predictive AI, you can reduce the chances of damage while sharpening the vision to identify if it happens.
6. Autonomous vehicle
Autonomous vehicles are similar to level 2 or level 3 self-driving cars (level here denotes the level of automation and human intervention, level 5 being the highest). These vehicles are best suited for the logistics industry—both in fleet management and in-warehouse transportation.
Here is how these vehicles work. These vehicles come embedded with a combination of technology—sensors, LIDAR and RADAR, GPS, cams, artificial intelligence, and other computer programs. LIDAR and RADAR are to bounce light and radiation, which is for object detection. The camera is to capture the path and surroundings while GPS and base maps are for route guidance. AI is to garner these inputs, synthesize them, and guide the actuator on the right path—basically acts like a programmed brain.
Autonomous vehicles can replace forklifts and carry trucks that move stocks in and out of the inventories. They might still require people to safely operate. Overall, this use case lowers safety incidents, reduces labor workload, and improves process efficiency.
Similarly, these vehicles can also power transportation and last-mile deliveries. Being semi-automated, might require an in-charge in place but limit their roles, freeing them to perform in-truck tasks and operate longer hours than usual for timely deliveries.
📖 Recommended read: Artificial intelligence decision making
7. Resource management
This includes the optimal utilization of equipment, fleet, storage spaces, docks, and finally workforce. Each of these has specific challenges and limitations that AI can address.
Firstly, workforce management. By forecasting and planning demand ahead through ML-based forecasting methods, one can employ the right number of operational staff needed for each section. This way, you avoid overstaffing and understaffing situations and reduce labor costs thereby.
This same is applicable to fleet management to predict how many orders need to be fulfilled and when. This can be most beneficial for logistics companies that rely on third-party fleet operators.
Dock utilization is another complicated area of inventory units. Dock is the loading and unloading unit of inventories. The challenge here is to match the dock with the right trucks so products can be loaded/unloaded in the most efficient manner (considering the distance between the dock and the relevant SKU) without making trucks wait longer. Multiple variables are considered here—when the truck arrives, time taken to load/unload, labor required, product categories, etc., based on this data, inferences can be generated on how long a truck loads/unloads stocks, how much labor is required to handle the operation, and which dock is situated closest to the stock keeping unit where products are stored/released from.
All of this helps the warehouse manager to streamline the complicated task, allowing the right amount of time and labor to it.
AI-based use cases also work for cross-docking utilization, a technique where warehouse operators unload consignments, sort, repack, and send them right away to fulfillment centers without storing them. Accurate demand forecasting combined with real-time truck monitoring empowers you to strategize this and perform at better efficiency.
8. Fraud detection
Given the number of goods handled and transferred, intruders and fraudsters are common in logistics companies’ premises. The traditional approach is physical security and CCTV cams which may not be effective 24/7. However, AI can introduce a more proactive approach.
AI-based intrusion detection works on deeper levels by analyzing inputs captured by live cams to identify potential intrusion activities. Through advanced tech like deep learning and facial recognition, the system can also identify the shape and characteristics of the object for more accuracy and fewer to null false positives.
These video analytics systems can instantly find this object of interest and alert the respective team through alarms and notifications for further action.
Similarly, these intrusion detectors can also be used for in-campus surveillance to detect the presence of unauthorized vehicles through automated vehicle number plate scanning and be immediately alerted.
Conclusion
The logistics industry has many ways to deal with the above-mentioned use cases through traditional methods. Artificial intelligence, however, becomes the most suitable solution due to the computational complexities around these logistics challenges.
With so many uncertainties wound around this industry, artificial intelligence brings in the certainty and resilience required to sustain and thrive comfortably. Being an industry where people are at the forefront in every part of its lifecycle, AI-based technologies can be the connecting power of it. From inventory shrinkage issues to quality control to sustainability, AI can address most of the problems the logistics industry faces.
How datakulture can help? We can help you find the optimal AI and ML-based solutions from tons of logistics analytics use cases, depending on your goals, challenges, and most importantly data, communicating limitations ahead.
We will be your trusted data partner getting the model developed, trained, and deployed, till it becomes adaptive. Send us your requirements using the Contact form.