6 ways to improve quality control in manufacturing
Quality control in manufacturing is all about preventing defects and meeting standards. Find out easy ways to improve quality control processes in your company to increase acceptance rates and customer satisfaction.

Ram
Nov 22, 2024 |
9 mins
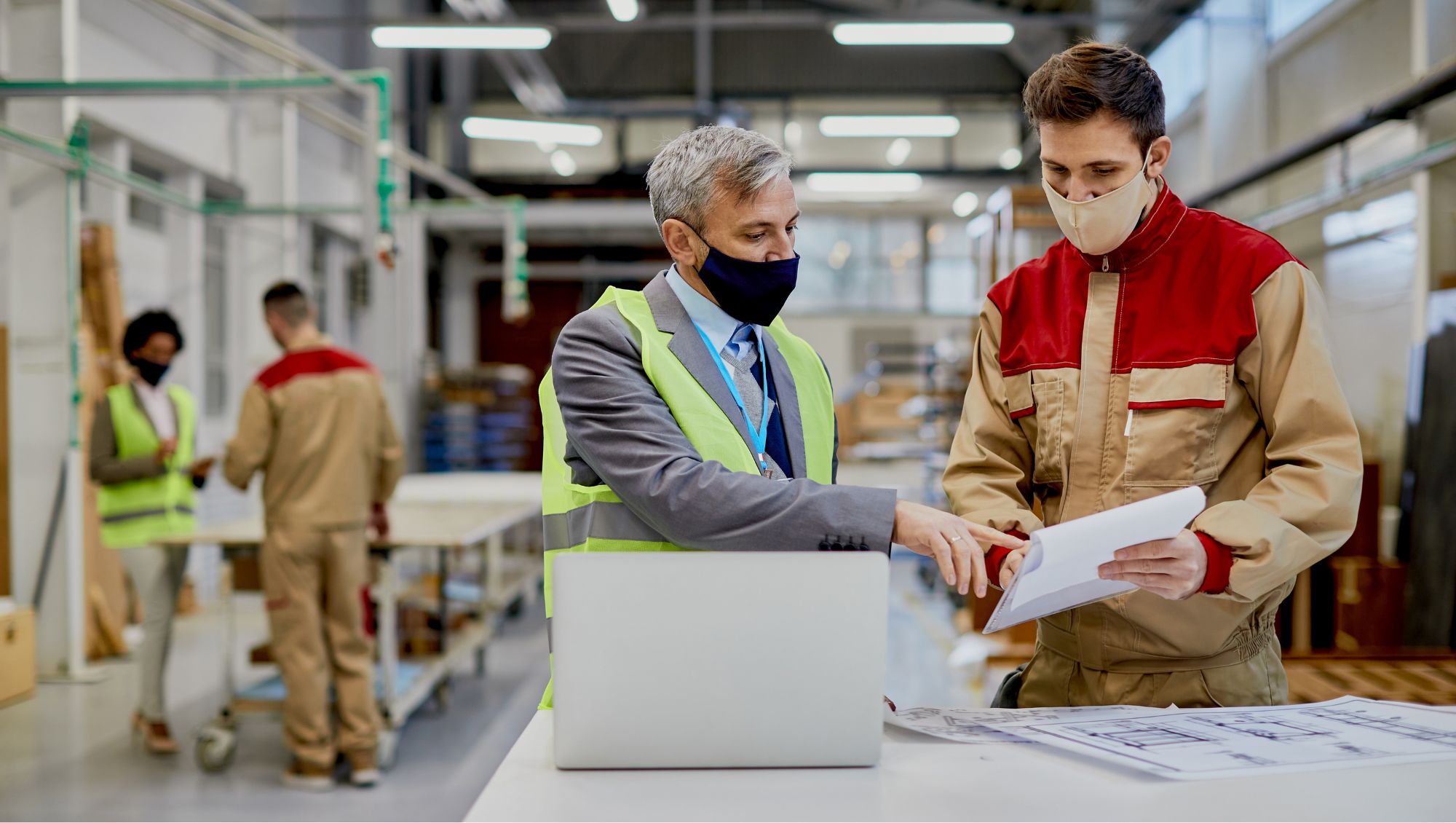
What is quality control in manufacturing?
Quality control in manufacturing involves checking materials, production process, and finished goods to maintain quality consistency and deliver high-quality products to customers. Example: A car spare parts manufacturer subjects every brake wire through different levels of testing to ensure it’s safe and reliable.
Quality assurance vs. quality control
The difference between quality assurance (QA) and quality control (QA) is that QC focuses on finding and correcting defects, whereas QA is concerned with improving processes and preventing quality issues.
The scope of QC applies to the finished product or production parts, but QA applies to the entire production process.
Benefits of quality control
Ensures that only high-quality products leave the unit and brand reputation among customers.
Helps the manufacturing company increase profits by reducing waste and defects.
Allows process improvement where repeated mistakes are avoided.
Helps with compliance adherence and standardization which can prevent hefty fines or recalls.
Quality control opens the door for cost savings by reducing wastes and fixing issues early.
Ways to improve quality control in manufacturing
1. 100% Inspection method
The 100% inspection method involves checking every product in a batch to see if they meet quality standards. It helps to ensure that there are no defects in the production line. 100% inspection is a crucial activity for industries like healthcare, aerospace, and automotive.
But extensive testing and inspection doesn’t come easy to all manufacturers for various reasons—meeting delivery deadlines, cost or labor constraints, and many similar challenges. In such cases, here’s how QC teams can implement a 100% inspection method.
Vision systems: setting up cameras, trackers, and IoT sensors paired with AI systems. The computer vision or sensor will capture images from all dimensions, whereas sensors can gather weight, size, or temperature during production. The AI system analyzes this collected data and looks for misalignments, visible defects, scratches, or any deviation in the product specifications.
Robotics for precision: use robotic arms set up with camera and inspection tools to inspect hard to reach areas of products.
Automated alerts: Any anomaly detected, send instant alerts for further inspection.
AI-Powered analytics: powering data analysis using AI to uncover patterns that can prevent defects before they arise.
Automated reporting: facilitating real-time data collection and reporting to reduce manual work and receive reports even when you aren’t at work.
2. Statistical process control
Statistical process control (SPC) is the use of statistical tools to track and measure if quality standards are being met. SPC is a bit opposite to 100% inspection as it focuses on quality improvement every step. Hence, this is suitable for high-volume production, where inspecting every product isn’t possible.
Here’s how you can implement statistical process control:
Charts, graphic tools, and visualization to display the system's stability right now.
Automatic identification of anomalies and process variations and why it occurs. Is it because of natural instabilities? Or does it appear too unusual?
Ensures the process stays within control limits, which are set based on historical data.
Identify chances for improvement to reduce defects and waste.
The major benefit of SPC is that it flags quality issues before it raises, while reducing the possibility of waste and reworks.
Let’s consider a beverage manufacturing company on how they use statistical process control to track the volume of the drink in each bottle. If the volume falls below the threshold for more than 10 to 15 bottles, the system alerts the monitoring team to correct the team. This prevents redoing a whole batch and saves time for everyone.
3. Statistical quality control
Statistical process quality is about tracking and controlling the manufacturing process, but statistical quality control (SQC) is about evaluating the final quality of the manufactured product. SQC looks for defects in batches and determines the batch acceptability. Any findings from this stage are applied to the final product. You could call SQC the reactive quality control process with the scope of work limited to post-production.
For any company to set up statistical quality control, they will require historical manufacturing and QC data to assess product quality. If set up correctly, this could help small manufacturers maintain product quality, consistency, and reduce waste and rejections.
What is statistical quality control composed of?
Descriptive statistics and data processing to find out mean, median, variance, and standard deviation of manufacturing metrics. Example: measuring the mean circumference of the product to see how far the batch is away from the ideal measurement.
Control charts for tracking process performance over time.
Acceptance sampling, which involves assessing a random batch’s quality to accept or reject it.
Process capability analysis to ensure that products are produced consistently as per specified constraints.
4. Six sigma
Six sigma is a fact-based, data-driven quality management approach to reduce defects in manufacturing operations. The main goal of six sigma is to achieve perfection and reduce failures and rejections to 0.00034%. Six sigma applies the DMAIC principle which expands into the following.
D - Define, where you identify the problem, project goals, and customer requirements with the help from tools like project charter, voice of the customer, etc.
M - Measure, which involves collecting data to understand current performance and set benchmarks and baseline metrics. Quality professionals use tools like pareto charts, process maps, and data collection tools to perform this.
A - Analyze, to find out the root cause of defects using the data analysis process. Tools like hypothesis testing, root cause analysis, and fishbone diagrams are used here.
I - Improve, put forward solutions to address the identified root causes.
C - Control, to ensure that the established process follows the track and provide training to employees if necessary using documentation, audit tools, and control charts.
Example for six sigma: A car spare parts manufacturing company uses the DMAIC approach as they identify 10% of goods contained defects.
They collect manufacturing, supply chain, vendor, and customer data.
Analyze and find the root cause of defects, which is dust in the paint room.
They install air filters to reduce the dust and monitor until their defect rate reduces to less than 1%.
Quality professionals get six sigma certification to exhibit their expertise by using six sigma methods for quality assurance.
5. Lean manufacturing
Lean manufacturing (or) lean production focuses on how to increase manufacturing productivity and quality outcomes while reducing wastes. It focuses on eliminating 7 types of wastes like overproduction, waiting, transport, overprocessing, inventory, motion, and defects. Here’s how lean manufacturing reduces waste.
Process optimization and streamlining of workflows to reduce variability. Establishing standardized process
Kaizen methods and continuous improvement to review and refine processes and make at least 1% improvement.
Poka yoke as in mistake proofing where you use tools and processes that prevent defects and process loopholes.
Implement Just-in-production to use the right amount of raw materials and produce what’s needed without waste.
Use real-time inventory management tracking and predictive analytics to align production with future requirements.
These measures work together to shorten the time you spend figuring out tasks, allowing you to focus on improvements and production goals.
6. Monitor and improve
Once you set up either one of the above quality improvement measures, you will need tracking performance changes and intervene before any minor issue becomes major defects.
Here is what it a quality team should do for tracking and improving:
Use real-time dashboards and analytics solutions to know if your quality metrics are on the right path. For example, a pharma manufacturing unit uses dashboards to check lot acceptance rates and CAPA closure rates and see how much % from a certain batch is pending for approval. Read the entire story of how a pharma company improved its acceptance rate.
Loop in customer feedback and incorporate it back into the production to meet changing customer expectations.
Making room for continuous monitoring and improvement to reduce defect rates and increase acceptance rates.
Enabling collaborative working among manufacturing and quality teams to make seamless and transparent flow of communication.
Final thoughts
Unlike some other goals and objectives, quality control in the manufacturing industry cannot be a one-day, one-person, or one-team task. It’s an ongoing process that impacts the bottom line—revenue, customer satisfaction, and business reputation. The usage of quality control methodologies like process standardization, lean manufacturing, and Six Sigma can help to a great extent.
But, how do you make it go from great to unparalleled?
How to make the quality control process an interesting and easy-to-perform task, given that one must do it every day?
How to reach the highly evolved stage of quality standards - Quality 4.0 technology methodology, which integrates people, processes, and technology…
The key lies in using data-driven tools and analytics, which involves leaving your major decisions to insights based on historical and present data. It’s about going beyond traditional tools and statistical processes and replacing them with the Internet of Things and AI. Since quality improvement isn’t just about making products better but the whole ecosystem around it, the shift from traditional to modern, innovative practices becomes paramount.